Healthcare providers, EMR developers, and hospital administrators are exploring this very question. AI is no longer a futuristic concept in medicine. It’s already reshaping how EMR systems handle vast amounts of patient data, predict health outcomes, and streamline daily tasks. The goal is clear: improve patient care while easing the workload of medical professionals.
This article delves into how AI is revolutionizing Electronic Medical Records systems to meet modern challenges. You will learn about its role in enhancing decision-making, improving data accuracy, and addressing administrative bottlenecks. We will also look at real-world applications, challenges, and future trends that show how AI is more than just hype in healthcare.
What is AI in EMR Systems?
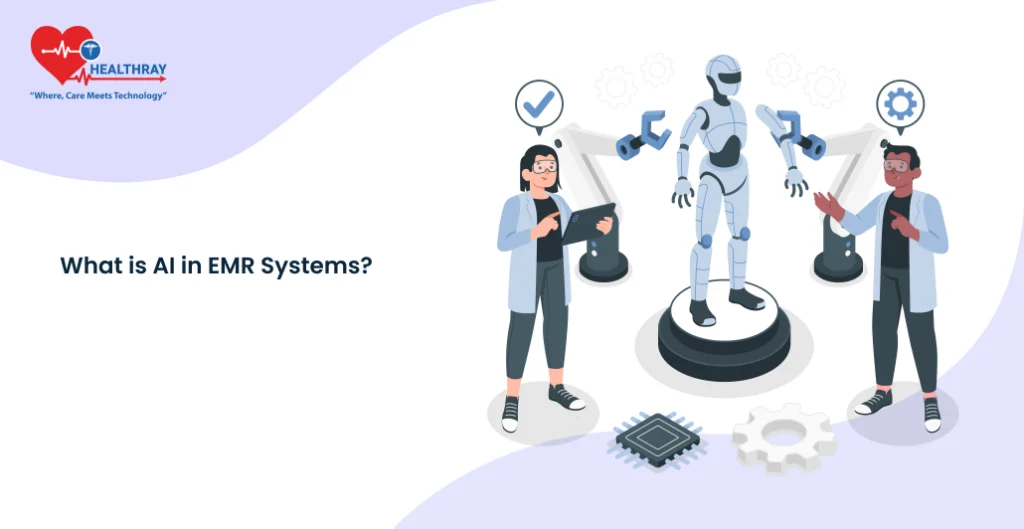
AI in EMR systems is the application of artificial intelligence technologies to improve how electronic medical records are managed, analyzed, and used. It involves machine learning, natural language processing, and predictive analytics to improve both patient care and operational efficiency.
NLP, for example, empowers EMR systems to be able to read and analyze unstructured data from physicians in the form of doctor’s notes or lab results to meaningful, actionable insights. In other words, machine learning finds patterns in patient data, which human providers would often miss, like the onset of chronic diseases early in the course.
By integrating AI, EMR systems evolve from static repositories of information to dynamic tools that actively assist healthcare professionals. Whether it is suggesting a diagnosis, flagging potential medication errors, or predicting patient outcomes, AI enhances the utility of EMRs in tackling everyday medical challenges.
Benefits of AI in EMR Systems
Artificial Intelligence is turning EMR systems smarter, faster, and more helpful for health professionals. Here’s how AI enhances EMRs:
Clinical Decision Support
AI algorithms analyze patient data to help in making critical decisions. For instance, they can flag abnormal lab results or suggest possible diagnoses based on a patient’s history. This reduces human error and ensures that healthcare providers are better equipped to offer precise treatments.
Improved Data Accuracy and Integrity
Maintaining accurate and complete patient records is essential. AI tools can identify inconsistencies, fill gaps in data, and even alert providers to potential errors. This improves not only record-keeping but also compliance with healthcare regulations.
Automation of Administrative Tasks
Documentation is one of the major time wasters for health professionals. AI-based applications automate tasks such as updating patient records, coding insurance claims, and scheduling appointments. This saves time and enables providers to spend more time on patient care.
Personalized Patient Care
Predictive analytics use both historical and real-time data to generate customized treatment plans. AI can identify those at risk of developing diseases such as diabetes or heart disease and suggest preventive interventions. This approach ensures better outcomes for patients and reduces costs in the long run.
Enhanced Interoperability
AI bridges gaps among various EMR systems. It is easier to share and retrieve data across organizations because of interoperability. This, in turn, improves coordination among healthcare teams, resulting in better care continuity.
Addressing Modern Medical Challenges with AI
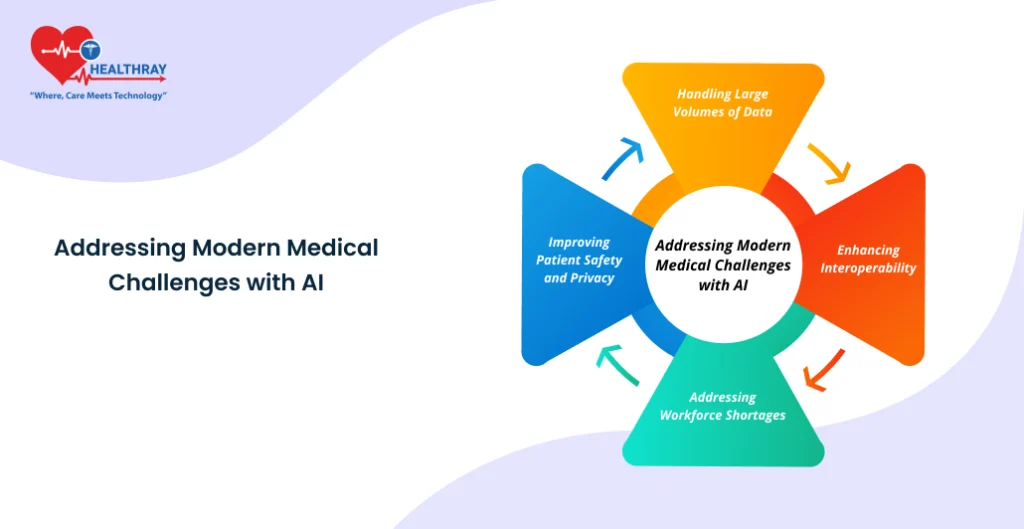
Modern day healthcare systems are facing escalating demands-from managing large-scale datasets and ensuring smooth care across multiprovider teams-to name a couple. AI-powered EMR is rising to the challenge on all these fronts.
Handling Large Volumes of Data
Modern medicine generates an unbelievable amount of patient data from lab results to imaging scans. AI tools can process and analyze this data at lightning speed, allowing healthcare providers to extract meaningful insights without delays. This is an important capability in identifying patterns, predicting disease outbreaks, and streamlining care plans.
Enhancing Interoperability
AI makes it easier for different EMR systems to communicate with one another. By translating and organizing data from various sources, it ensures that critical patient information flows seamlessly between departments, clinics, and hospitals. This reduces errors and ensures patients receive coordinated care, even in complex settings.
Addressing Workforce Shortages
Staff shortages are plaguing the health care industry, and most of the time administrative tasks consume valuable time. AI automates routine tasks like documentation and appointment scheduling so that medical staff may have more time to give attention to patients. The efficiency reduces stress and makes full use of existing teams.
Improving Patient Safety and Privacy
AI-driven EMR systems are also crucial in protecting sensitive data. They identify unusual access patterns or vulnerabilities that can enhance data security and help meet stringent compliance standards. Additionally, AI-powered alerts can flag unsafe medication combinations or dosages, which directly protects patient safety.
Challenges in AI-EMR Integration
Although the possibility of AI in EMR systems is undeniable, there are challenges with the adoption of these technologies. All these barriers have to be addressed for the successful implementation of these technologies.
Data Privacy and Security Concerns
Information belonging to a patient is very confidential. Adding AI to systems may raise compliance issues toward such regulations as HIPAA. Data security is fundamental; breaches have severe results not only for patients but for organizations, too. In AI, the security framework will have to be aligned more toward encryption, access control, and anomaly detection for data safety.
Integration with Legacy Systems
Most healthcare facilities work with older EMR systems that were never designed taking into consideration the advent of AI. To upgrade these or make sure that they integrate well with new AI-based solutions is expensive and takes a lot of time. EMR platforms, therefore, also are not standardised.
Resistance to Adoption
Healthcare providers and administrators may resist the adoption of AI due to reliability, training, and disruption in workflow. Overcoming such resistance requires building trust in AI capabilities and adequate support during implementation.
Cost and Implementation Barriers
The implementation of AI often involves significant investment in technologies, infrastructure, and human resources. Small practices and hospitals with limited budgets could find it difficult to sustain these costs without clear evidence regarding long-term ROI.
Ethical and Legal Challenges
AI systems are only as good as the data they are trained on. Biases in historical data can lead to unequal treatment or inaccurate predictions. Another question that comes up when an AI system makes a wrong or harmful recommendation is one of liability.
Best Practices for Implementing AI in EMR Systems
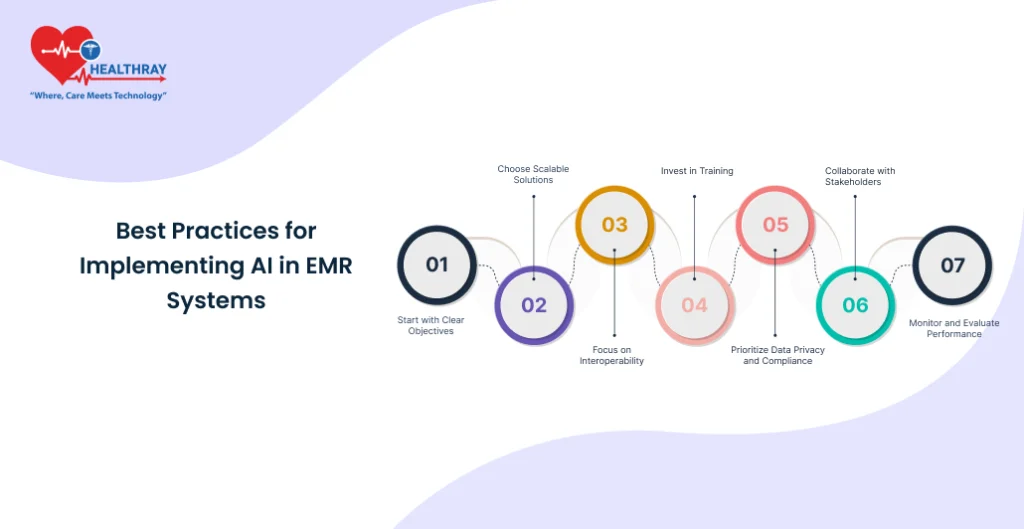
Implementing AI-Powered EMR Systems successfully calls for careful consideration so that it aligns with the needs of healthcare providers and administrators, minimizing disruption.
Start with Clear Objectives
Identify specific goals of AI integration: reduce administrative workload, improve clinical decision-making, or enhance data accuracy. Clear objectives ensure that technology aligns with organizational priorities and patient care outcomes.
Choose Scalable Solutions
AI solutions should be malleable enough to meet the changing needs of a healthcare facility. Scalable platforms make it easy to expand, whether it’s adding new AI functionalities or accommodating more users and data.
Focus on Interoperability
Ensure that the AI tools you choose are fully compatible with existing EMR systems and other healthcare software. This minimizes the risk of data silos and makes workflows across departments and organizations smoother.
Invest in Training
Comprehensive training programs are essential for healthcare providers, administrators, and IT staff. Familiarity with AI features and confidence in using them is crucial for adoption. Ongoing support and user-friendly interfaces also encourage engagement.
Prioritize Data Privacy and Compliance
Protect the patient data with strong safeguards that will include encryption, role-based access controls, and ensuring compliance with regulations such as HIPAA. Periodic audits and updates will maintain the effectiveness of security measures against new threats.
Collaborate with Stakeholders
All key stakeholders, including physicians, nurses, administrators, and IT teams, should be engaged in the process early on to tailor the AI system according to real-world challenges, thereby easing the transition process.
Monitor and Evaluate Performance
Monitor key metrics including administration tasks saved time, reduced errors, and the actual rate of user satisfaction; an organization will get improved outcomes by refining the process on regular evaluation over AI implementation.
Case Studies: AI in Action
Real-world examples highlight how AI integration in EMR systems is transforming healthcare operations and patient outcomes. Here are two notable cases that showcase the potential of AI in action.
Streamlining Administrative Work in a Multi-Specialty Clinic
A multi-specialty clinic implemented an AI-assisted EMR system to reduce its physicians’ documentation workloads. In consultation, the system utilizes NLP to transcribe and format patient interactions directly into EMRs.
Results:
- Administrative time for physicians dropped by 30%.
- Patient satisfaction scores improved due to more face-to-face interaction with doctors.
- Billing accuracy increased as AI tools auto-coded patient visits for insurance claims.
Enhancing Predictive Analytics in a Regional Hospital
A regional hospital implemented an AI module in its existing EMR to predict patient readmissions. Using historical data and machine learning, the system flagged patients at high risk for complications post-discharge. This allowed care teams to design personalized follow-up plans.
Results:
- Hospital readmission rates decreased by 18%.
- Nurses reported a 20% improvement in workflow efficiency as AI suggested targeted interventions.
- The hospital saved significant costs associated with avoidable readmissions.
Reducing Medication Errors in a Large Healthcare Network
A large healthcare network integrated an AI-powered tool to analyze prescriptions and medication orders in real time. The tool flagged potential errors, such as drug interactions or incorrect dosages, before they reached patients.
Results:
- Medication errors reduced by 40%.
- Provider trust in the system grew due to consistent accuracy.
- Compliance with safety regulations improved across all locations.
Future Trends in AI and EMR Systems
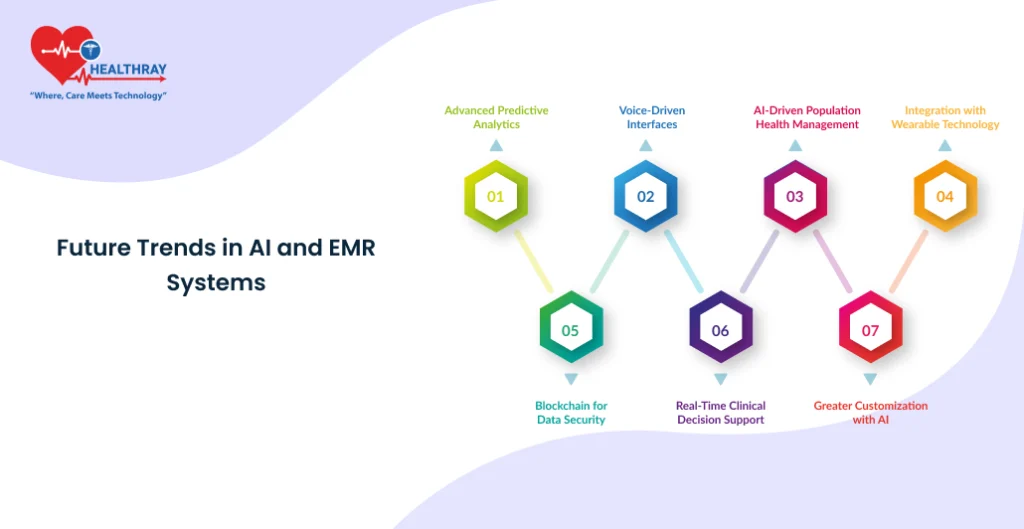
It just starts there. The integration of AI into EMR systems marks the beginning. New and emerging technologies coupled with ever-changing healthcare needs shape the future of such systems. Here’s a look at trends to watch:
Advanced Predictive Analytics
AI will continue refining its ability to predict health outcomes. Future EMR systems might be using patient data combined with environmental or genetic information to forecast illnesses before they manifest, which allows for interventions even earlier than today.
Voice-Driven Interfaces
Voice recognition technology will soon become more accurate and widespread in EMR systems. Physicians will soon depend on voice commands to enter data, retrieve patient records, and even generate reports, reducing the need for manual entry.
AI-Driven Population Health Management
AI will enhance the ability of EMRs to analyze data across populations, identifying trends and risks for specific communities. This may revolutionize public health initiatives by focusing resources on where they are needed most.
Integration with Wearable Technology
The future of EMRs involves seamless integration with wearable devices. Data from smartwatches, fitness trackers, and other health-monitoring tools will feed directly into EMR systems, providing real-time insights for both patients and providers.
Blockchain for Data Security
AI and blockchain technologies are likely to join forces to strengthen data security. Blockchain can ensure that patient data remains tamper-proof while AI analyzes access patterns and flags potential breaches.
Real-Time Clinical Decision Support
AI-powered EMRs will deliver actionable insights during consultations, such as suggesting tests, alerting providers to rare conditions, or flagging alternative treatment plans—all in real-time.
Greater Customization with AI
Future EMR systems will adapt to individual user preferences. AI will tailor interfaces for different roles, whether it’s a nurse, administrator, or specialist, improving efficiency and user satisfaction.
Conclusion
AI is revolutionizing the way EMR systems have approached modern medical issues. With enhanced clinical decision making, better data, and fewer administrative burdens, AI turns Hospital Management System from rather ordinary tools into high-functioning instruments for use by healthcare providers, developers, and hospital administrators.
However, data privacy issues, integration into legacy systems, and training staff in the new changes are important barriers that have to be overcome to implement these practices successfully. But following the best practices, learning through real examples makes the implementation smoother and more impactful.
The future is bright, promising tremendous possibilities with the marriage of AI with voice recognition technology, wearables, and blockchain. By embracing such innovations, healthcare organizations are better positioned to ensure a prepared future while making excellent patient care possible in the present.