Artificial Intelligence (AI) is reshaping industries, and healthcare is no exception. One area where AI is making significant strides is in Electronic Medical Records (EMR) systems. EMRs are essential for storing, managing, and analyzing patient data, but integrating AI into these systems can take their utility to the next level. From improving diagnostic accuracy to personalizing patient care, AI has the potential to revolutionize outcomes.
But how does AI fit into the world of EMRs? What are the tangible benefits, and what challenges should be expected along the way? This post will explore these questions, unraveling the transformative power of AI in EMR Softwares. We’ll also discuss strategies for successful implementation, backed by real-world examples.
Understanding AI in EMR Systems
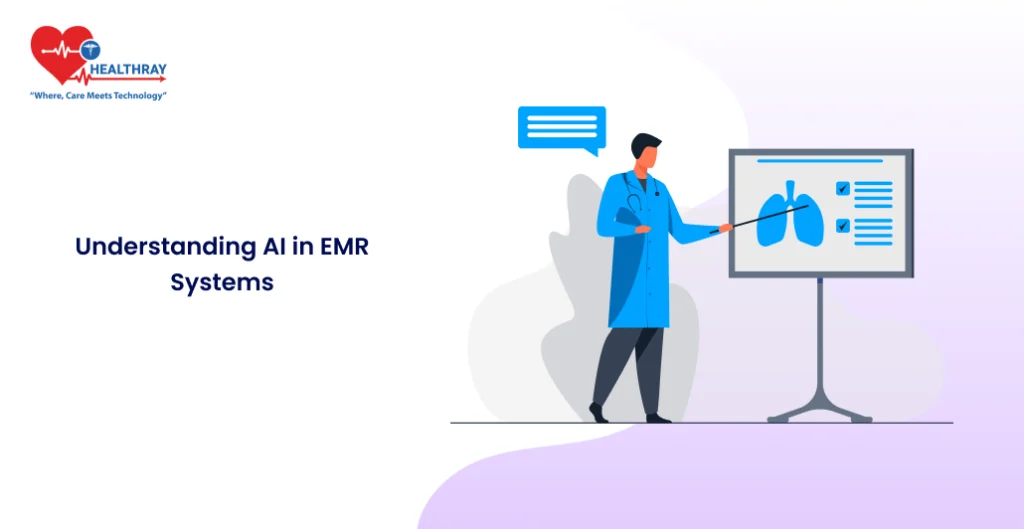
AI in EMR systems refers to the integration of advanced algorithms and machine learning models into electronic records to analyze and process healthcare data more effectively. This collaboration helps streamline workflows, identify patterns in patient health trends, and offer insights that traditional systems cannot.
Key Components of AI in EMR
- Data Analytics: AI leverages patient data to detect trends, predict outcomes, and suggest interventions. This could mean identifying high-risk patients or optimizing care plans based on historical data.
- Natural Language Processing (NLP): With NLP, AI can analyze unstructured data such as physician notes, prescriptions, and lab results, transforming them into actionable insights.
- Decision Support Systems: AI-driven decision support provides clinicians with recommendations, helping improve accuracy in diagnostics and treatment planning.
- Predictive Modeling: Predictive algorithms help forecast patient conditions, such as potential readmissions or the likelihood of developing chronic illnesses.
Current Trends in AI and EMR Integration
- Remote Patient Monitoring: AI-enabled systems are analyzing real-time patient data collected from wearable devices to provide proactive care.
- Interoperability Advancements: Efforts are underway to make AI tools more compatible with diverse EMR platforms, ensuring seamless data sharing.
- Regulatory Compliance: AI tools are being fine-tuned to meet healthcare compliance standards, ensuring patient safety and data privacy.
Benefits of AI-Integrated EMR Systems
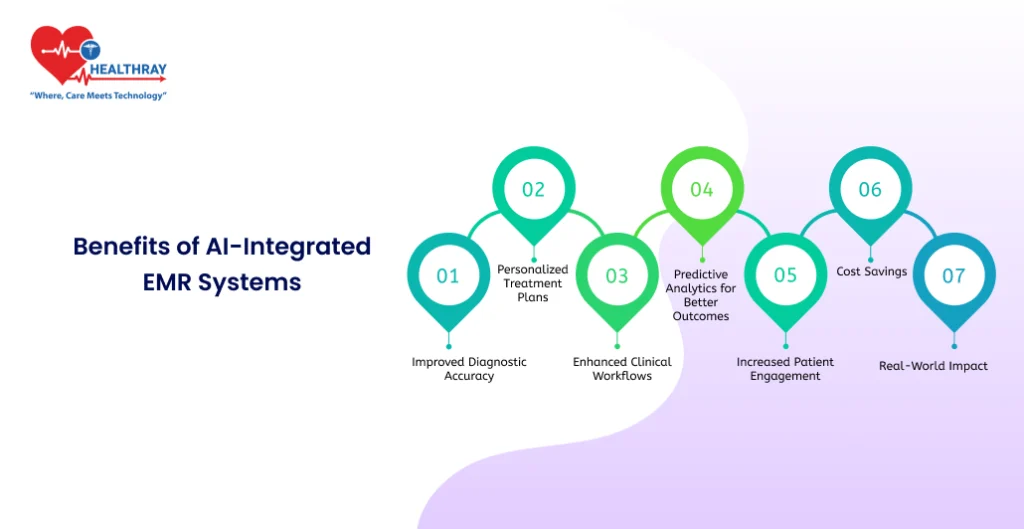
AI-enhanced EMR systems offer a wide range of advantages for healthcare professionals and patients. By addressing inefficiencies and providing actionable insights, these systems are transforming the way care is delivered.
Improved Diagnostic Accuracy
AI tools analyze vast datasets to identify patterns and correlations that may not be apparent to human clinicians. This can lead to earlier and more accurate diagnoses, reducing misdiagnosis rates. For instance, AI can flag anomalies in lab results or imaging scans that suggest potential health issues.
Personalized Treatment Plans
Every patient is unique, and AI helps tailor treatments to individual needs. By analyzing a patient’s medical history, lifestyle, and genetic data, AI can recommend treatment options that are most likely to succeed. This approach boosts patient satisfaction and outcomes.
Enhanced Clinical Workflows
Administrative tasks like documentation and data entry can be burdensome for healthcare providers. AI-powered EMR systems automate these processes, giving clinicians more time to focus on patient care. Tools like voice recognition for charting and automated appointment scheduling simplify day-to-day operations.
Predictive Analytics for Better Outcomes
AI uses predictive models to anticipate potential health complications. For example, an AI-integrated EMR system might predict the likelihood of a hospital readmission or complications following surgery, enabling providers to intervene proactively.
Increased Patient Engagement
With AI, patients can access personalized health recommendations and real-time updates on their conditions through EMR-linked portals or apps. This encourages patients to take an active role in managing their health.
Cost Savings
By improving efficiency and reducing errors, AI-powered EMR systems help healthcare organizations save money. From optimizing resource allocation to preventing unnecessary tests, AI ensures that operational costs are kept in check.
Real-World Impact
Healthcare institutions adopting AI-driven EMRs have reported reduced readmission rates, faster diagnosis times, and higher patient satisfaction. These tangible results highlight the value of integrating AI into Electronic Medical Record Systems.
Implementation Strategies for AI in EMR Systems
Integrating AI into EMR systems requires thoughtful planning and execution. A successful implementation not only enhances patient outcomes but also ensures that healthcare organizations can maximize the potential of these advanced tools.
Assess Organizational Readiness
Before implementing AI, evaluate the current state of your EMR system and overall technological infrastructure. This includes:
- Assessing data quality and storage systems.
- Identifying gaps in interoperability between existing tools and AI platforms.
- Gauging the readiness of staff to adopt new technologies.
Develop a Clear Roadmap
A step-by-step approach is essential for integrating AI into EMR systems. This roadmap should include:
- Setting measurable objectives (e.g., reducing diagnostic time by 20%).
- Defining timelines for implementation phases.
- Establishing benchmarks to evaluate progress.
Train and Support Healthcare Staff
One of the most critical aspects of AI adoption is ensuring that healthcare professionals are comfortable using the new tools. Provide training sessions to familiarize staff with AI capabilities, workflows, and troubleshooting. Create a support system to address concerns and ensure a smooth transition.
Prioritize Data Privacy and Security
AI in healthcare requires access to sensitive patient data. To protect this information:
- Implement robust encryption protocols.
- Ensure compliance with regulations like HIPAA (Health Insurance Portability and Accountability Act).
- Regularly audit data management practices to identify vulnerabilities.
Start Small with Pilot Programs
Instead of overhauling your entire EMR system, start with a pilot program to test AI integration. Choose a specific department or use case, such as automating chart reviews or implementing predictive analytics for chronic disease management. Use the results to refine and expand the system.
Collaborate with AI and EMR Vendors
Work closely with technology providers to customize solutions for your organization. Ensure that the chosen tools align with your workflows, clinical needs, and compliance requirements.
Monitor Performance and Adjust
After implementation, continuously monitor the system’s performance to ensure it meets your objectives. Collect feedback from staff, track key metrics, and adjust workflows or tools as needed.
Challenges and Considerations for AI in EMR Systems
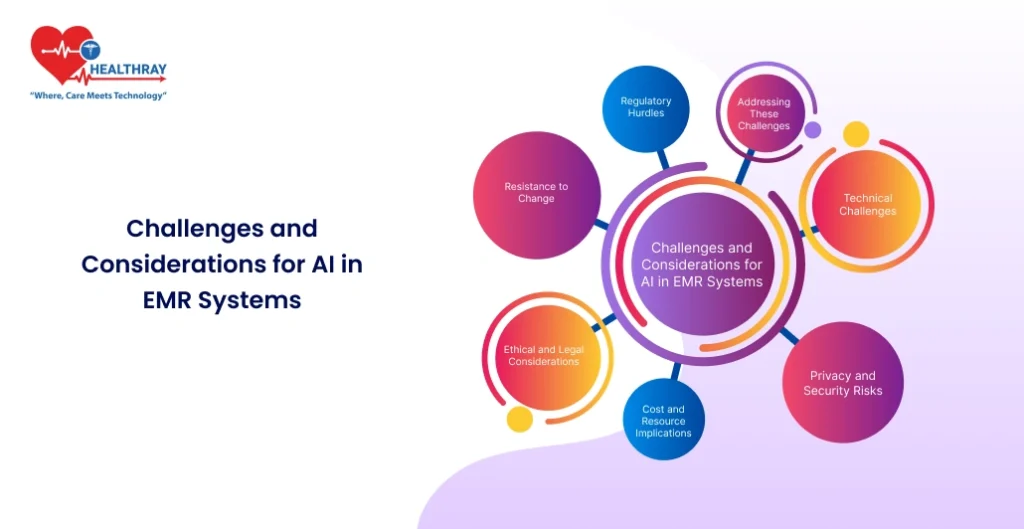
While the benefits of integrating AI into EMR systems are clear, the process comes with its own set of challenges. Addressing these issues proactively is critical for a smooth and successful implementation.
Technical Challenges
- Interoperability Issues: Many EMR systems lack compatibility with advanced AI tools. Ensuring seamless integration across platforms and devices requires significant effort.
- Data Quality Concerns: AI systems depend on clean, structured, and comprehensive data. Inconsistent or incomplete patient records can limit AI’s effectiveness.
- Scalability: As AI systems grow in complexity, maintaining performance and processing speed becomes a challenge, especially for large healthcare networks.
Privacy and Security Risks
Patient data is highly sensitive, and integrating AI increases the number of potential vulnerabilities. Key concerns include:
- Unauthorized access to EMR data.
- Potential misuse of AI tools, such as biases in predictive algorithms.
- Ensuring compliance with healthcare privacy regulations like HIPAA and GDPR.
Cost and Resource Implications
AI implementation can require significant upfront investment, including:
- Purchasing or upgrading hardware and software.
- Training staff to use AI tools effectively.
- Allocating resources for ongoing system maintenance and updates.
Ethical and Legal Considerations
- Bias in AI Models: AI algorithms may unintentionally replicate biases present in training data, leading to unequal outcomes for patients.
- Accountability: Determining responsibility for AI-driven decisions, especially in high-stakes medical situations, can be complex.
- Informed Consent: Patients may need reassurance and transparency about how their data is used in AI-driven EMR systems.
Resistance to Change
Healthcare professionals may be hesitant to adopt AI tools due to:
- Concerns about job displacement or de-skilling.
- Fear of over-reliance on technology at the expense of clinical judgment.
- Uncertainty about the long-term reliability of AI systems.
Regulatory Hurdles
Governments and healthcare organizations impose strict regulations on EMR systems. Navigating these rules while integrating AI can be a time-consuming process.
Addressing These Challenges
To mitigate these obstacles:
- Invest in robust training programs to prepare staff.
- Partner with trusted vendors to ensure system compliance and security.
- Conduct regular audits to evaluate AI system performance and fairness.
- Start small with low-risk pilot programs to build confidence in AI tools.
Future Outlook for AI in EMR Systems
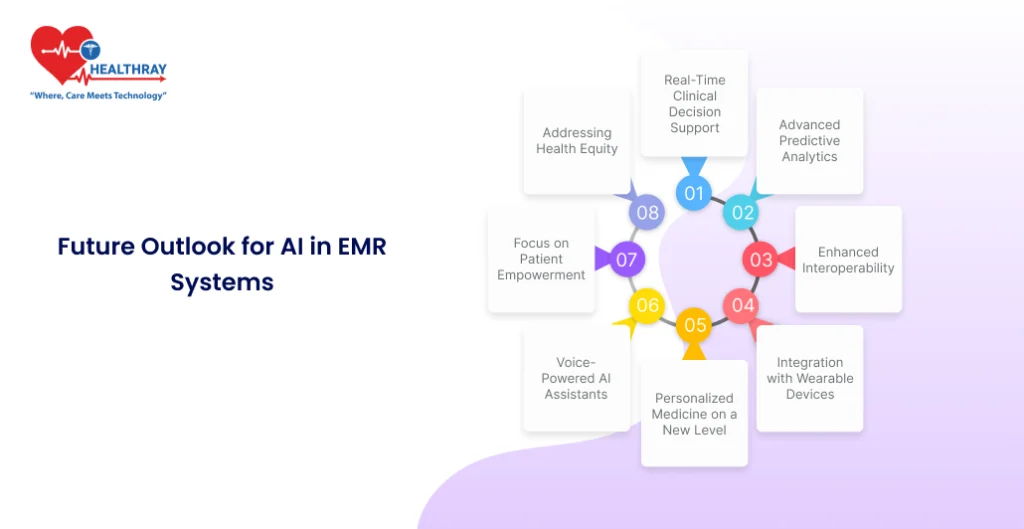
The role of AI in EMR systems is expected to expand significantly in the coming years. Emerging trends and innovations will continue to shape how healthcare organizations leverage AI to improve efficiency and outcomes.
Real-Time Clinical Decision Support
AI will increasingly provide real-time insights during patient consultations. For instance, EMR systems will suggest diagnoses, recommend treatments, and flag potential complications based on live patient data.
Advanced Predictive Analytics
Predictive models will evolve to anticipate not only individual patient risks but also broader trends such as disease outbreaks. This will enable healthcare providers to prepare resources and interventions proactively.
Enhanced Interoperability
Future AI tools will focus on breaking down silos within healthcare systems. Seamless data sharing between different EMR platforms and healthcare providers will become standard, fostering better collaboration and patient care.
Integration with Wearable Devices
AI will process data from wearable health technologies, such as fitness trackers and smartwatches, directly within EMR systems. This integration will provide a more comprehensive view of a patient’s health and support preventative care measures.
Personalized Medicine on a New Level
AI will delve deeper into genomics and patient-specific data to offer hyper-personalized treatment plans. This could include custom drug therapies or tailored lifestyle recommendations based on a patient’s unique genetic makeup.
Voice-Powered AI Assistants
The use of voice recognition and AI-powered virtual assistants will continue to grow. These tools will make it easier for clinicians to interact with EMR systems hands-free, improving efficiency during patient consultations.
Focus on Patient Empowerment
AI in EMR systems will enable patients to access more actionable insights about their health. Predictive tools and tailored wellness recommendations will empower patients to make informed decisions about their care.
Addressing Health Equity
Future AI systems will emphasize fairness by reducing biases in healthcare delivery. AI developers are already working on models that ensure equitable care for diverse populations.
Conclusion
The integration of AI into EMR systems is revolutionizing healthcare by improving efficiency and patient outcomes. While challenges exist, the potential benefits—such as personalized care, predictive analytics, and streamlined workflows—make it a transformative innovation. For healthcare organizations, investing in AI-driven Hospital Management Systems can pave the way for smarter, more responsive, and more patient-focused care.