Healthcare organizations constantly face challenges like managing patient data, ensuring accuracy, and streamlining workflows. Traditional Electronic Medical Record (EMR) systems helped digitize records, but they often added complexity instead of reducing it. This is where artificial intelligence (AI) steps in.
AI-powered EMR systems are transforming healthcare efficiency by automating routine tasks, providing actionable insights, and enhancing data security. They don’t just store information; they learn from it, ensuring faster, smarter decision-making.
In this article, we’ll explore the benefits these systems offer to healthcare administrators, clinicians, doctors, and IT professionals. By the end, you’ll know how these technologies can reduce administrative burdens, improve patient care, and support seamless system integration.
Understanding AI-Powered EMR Systems
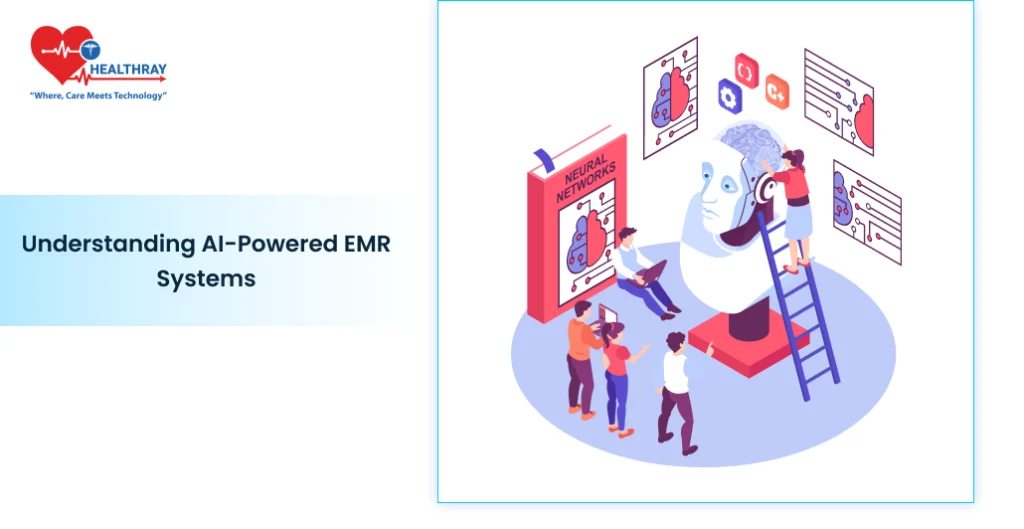
Electronic Medical Record (EMR) systems have long been a cornerstone of modern healthcare, offering a centralized platform for storing and managing patient data. However, integrating artificial intelligence (AI) into these systems takes their functionality to a whole new level.
What Are AI-Powered EMR Systems?
AI-powered EMR systems go beyond basic data storage. They analyze vast amounts of information, detect patterns, and assist in decision-making. These systems utilize machine learning algorithms, natural language processing (NLP), and predictive analytics to support healthcare professionals in their daily workflows.
For instance, AI can help identify potential diagnoses based on patient symptoms or flag abnormalities in test results. This capability reduces manual effort and ensures critical insights aren’t missed.
Key Components of AI Integration
- Data Analysis: AI identifies trends in patient data, such as recurring health conditions or anomalies in lab results, providing actionable insights.
- Natural Language Processing (NLP): Converts unstructured notes from doctors into structured data, making it easier to analyze and share.
- Predictive Analytics: Forecasts patient outcomes, helping clinicians make proactive treatment decisions.
- Automation: Streamlines repetitive tasks like appointment scheduling, billing, and report generation.
How AI Enhances EMR Systems
- Improved Accuracy: Reduces human error by cross-verifying data inputs.
- Real-Time Updates: Provides instant insights and alerts based on incoming data.
- Interoperability: Integrates seamlessly with other healthcare systems, ensuring a unified patient care approach.
This transformative technology not only aids healthcare professionals but also improves the overall patient experience by minimizing delays and enhancing care quality.
Benefits of AI-Powered EMR Systems
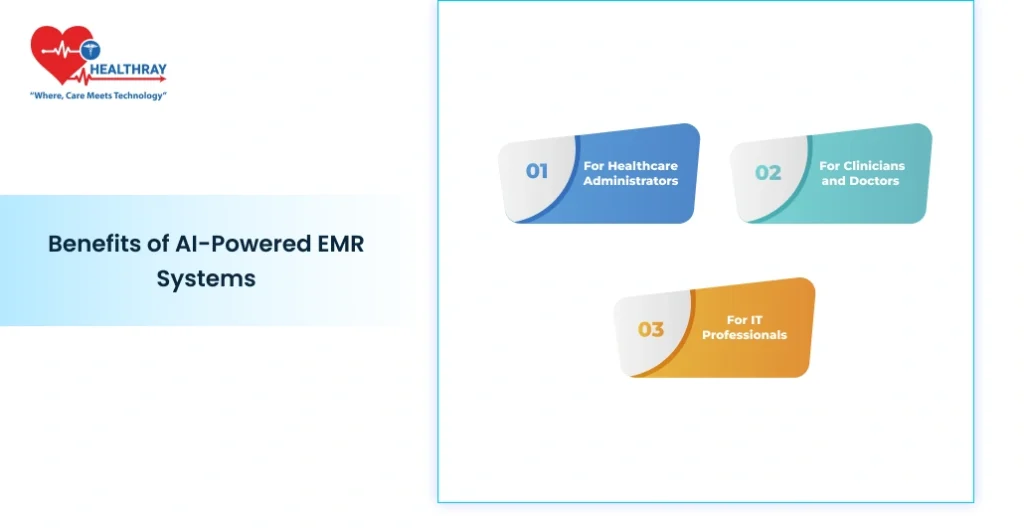
AI-powered Electronic Medical Record (EMR) systems bring significant advantages to healthcare professionals and organizations. Whether you’re a healthcare administrator, a clinician, or an IT professional, these systems offer tailored benefits to address your unique challenges.
For Healthcare Administrators
Healthcare administrators are often tasked with balancing operational efficiency, cost management, and patient satisfaction. AI-powered EMR systems simplify these responsibilities by providing data-driven insights and automation.
- Operational Efficiency: AI streamlines workflows, reducing bottlenecks in administrative processes like scheduling and billing.
- Cost Savings: Automation of routine tasks minimizes manual labor, saving time and resources.
- Enhanced Compliance: AI tracks regulatory changes and ensures documentation aligns with legal and compliance requirements.
For Clinicians and Doctors
Clinicians often face the burden of administrative tasks, which take time away from patient care. AI addresses this by automating repetitive duties and offering clinical decision support.
- Reduced Documentation Burden: AI-powered NLP converts voice dictation or handwritten notes into structured data, allowing clinicians to focus on patient interactions.
- Enhanced Decision-Making: Predictive analytics provides personalized treatment suggestions based on patient history and symptoms.
- Improved Patient Care: Real-time alerts for drug interactions, abnormal lab results, or follow-up needs ensure patients receive timely and appropriate care.
For IT Professionals
IT professionals in healthcare manage system integrations, data security, and technology upgrades. AI-powered EMR systems simplify these tasks with advanced tools and features.
- Seamless Integration: AI systems easily connect with other healthcare technologies, creating a unified ecosystem.
- Data Security: AI monitors for potential breaches and ensures compliance with privacy regulations like HIPAA.
- Scalable Infrastructure: These systems adapt to growing data volumes, making them future-ready.
Why These Benefits Matter
AI-powered EMR systems are more than just tools; they are enablers of better healthcare outcomes. By reducing errors, enhancing operational efficiency, and improving decision-making, these systems empower professionals at every level to work smarter and achieve better results.
Implementation Strategies for AI-Powered EMR Systems
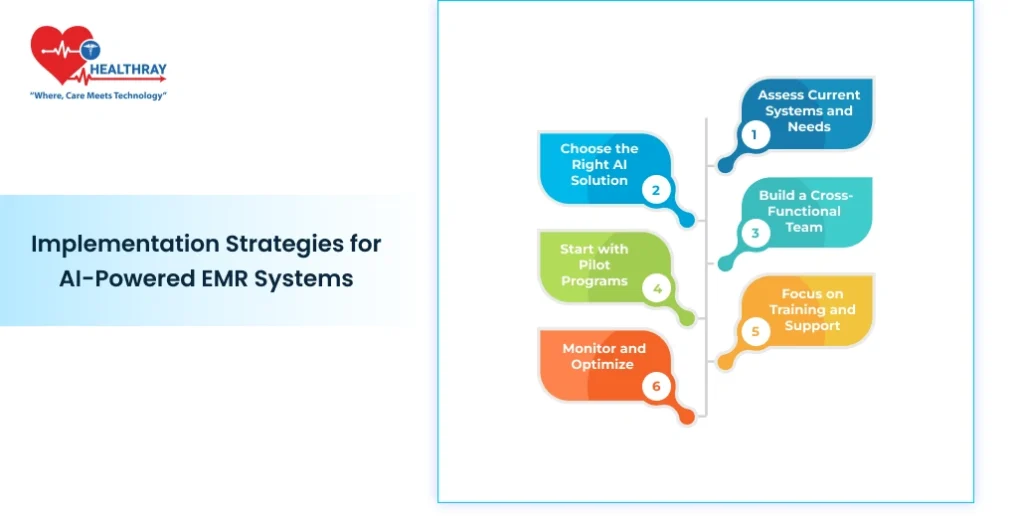
Integrating AI into existing Electronic Medical Record (EMR) systems can feel like a daunting task. However, with a structured approach, healthcare organizations can make this transition smoothly and efficiently.
Assess Current Systems and Needs
Start by evaluating the existing EMR system and identifying gaps that AI can fill. This ensures the integration focuses on areas that need improvement.
- Analyze Workflow Bottlenecks: Determine which processes, such as scheduling or data entry, consume the most time and resources.
- Set Clear Goals: Define what you want to achieve—better accuracy, faster workflows, or improved patient care.
Choose the Right AI Solution
Not all AI tools are created equal. Select a solution that aligns with your organization’s goals and integrates well with your current infrastructure.
- Scalability: Ensure the AI system can handle increasing data volumes and adapt to future needs.
- Compatibility: Check whether the solution integrates seamlessly with your EMR and other healthcare systems.
- Vendor Support: Choose a provider with a strong track record and robust support services.
Build a Cross-Functional Team
Successful implementation requires collaboration between various stakeholders.
- Healthcare Administrators: Oversee project timelines and budgets.
- Clinicians: Provide input on usability and workflow integration.
- IT Professionals: Manage technical aspects, including installation and data migration.
Start with Pilot Programs
A phased rollout minimizes disruptions and allows teams to adjust to the new system gradually.
- Select a Test Group: Choose a department or group of users to test the AI-powered EMR system.
- Gather Feedback: Monitor how the system performs and address issues promptly.
- Iterate and Improve: Refine the setup based on feedback before expanding the rollout.
Focus on Training and Support
A system is only as effective as its users. Invest in training to help staff understand and utilize AI-powered features.
- Comprehensive Training: Conduct workshops, provide manuals, and offer ongoing support to ensure everyone feels confident using the system.
- Dedicated Support Team: Have IT professionals available to resolve technical issues during and after implementation.
Monitor and Optimize
Once the system is fully implemented, continuous monitoring ensures it delivers on its promises.
- Performance Metrics: Track key indicators such as time savings, error rates, and user satisfaction.
- Regular Updates: Keep the AI algorithms updated to improve performance and adapt to evolving needs.
- User Feedback: Maintain open communication with staff to identify areas for further improvement.
By following these steps, healthcare organizations can smoothly transition to AI-powered EMR systems while minimizing risks and maximizing benefits.
Challenges and Solutions in AI Integration with EMR Systems
Integrating AI into Electronic Medical Record (EMR) systems can bring transformative benefits, but it also presents unique challenges. Addressing these hurdles head-on is key to successful implementation.
Data Privacy and Security Concerns
AI systems rely on extensive data to function effectively, but this raises concerns about data privacy and compliance with regulations like HIPAA.
- The Challenge: Ensuring patient data remains secure while allowing AI to analyze and process it.
- The Solution: Implement advanced encryption protocols and conduct regular security audits. Use AI algorithms that anonymize data during processing to enhance privacy without compromising functionality.
High Implementation Costs
Adopting AI-powered systems often requires significant upfront investment in technology, training, and infrastructure.
- The Challenge: Budget constraints for many healthcare organizations, especially smaller facilities.
- The Solution: Start with a scalable AI solution and roll it out in phases. Look for grants or partnerships with tech companies that offer subsidized solutions for healthcare providers.
Resistance to Change
Healthcare professionals, especially those accustomed to traditional workflows, may resist adopting new AI-powered systems.
- The Challenge: Fear of job displacement or the perceived complexity of AI systems.
- The Solution: Focus on training and emphasize how AI can enhance, not replace, human expertise. Share case studies showing how other organizations benefited from similar integrations.
Interoperability Issues
Integrating AI into existing EMR systems can lead to compatibility problems, especially in organizations using older software.
- The Challenge: Inconsistent data formats and lack of seamless communication between systems.
- The Solution: Choose AI solutions designed with interoperability in mind. Work closely with vendors to ensure the system integrates smoothly with legacy software.
Data Quality and Standardization
AI systems require high-quality, standardized data to function optimally. Inconsistent or incomplete records can hinder performance.
- The Challenge: Variability in data entry practices across different departments.
- The Solution: Implement strict data entry protocols and use AI tools that can clean and standardize data automatically.
Ethical Considerations
Using AI in healthcare raises ethical questions about decision-making, such as the risk of bias in AI algorithms.
- The Challenge: Ensuring AI recommendations are fair and unbiased.
- The Solution: Regularly audit AI systems for biases and use diverse datasets for training the algorithms. Involve ethics committees in the implementation process to address concerns transparently.
Ongoing Maintenance and Updates
AI-powered systems require consistent updates and maintenance to stay effective and secure.
- The Challenge: Allocating resources for long-term upkeep.
- The Solution: Partner with vendors offering robust post-implementation support and regular updates. Train IT teams to handle routine maintenance tasks internally.
Why Addressing These Challenges Matters
Proactively solving these challenges ensures that AI-powered EMR systems deliver their full potential. This not only improves operational efficiency but also strengthens trust among healthcare professionals and patients.
Case Studies: Real-World Applications of AI in EMR Systems
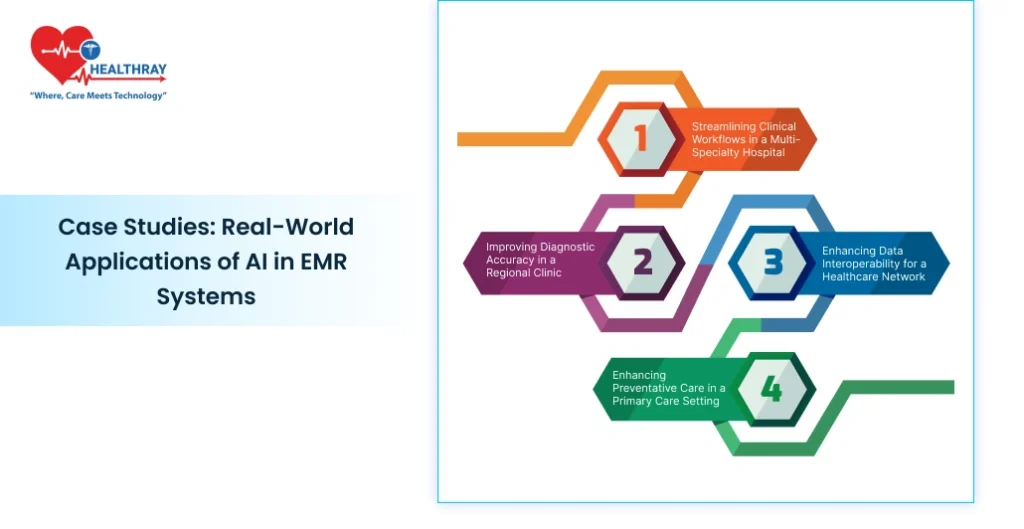
Real-world examples of AI-powered EMR implementations demonstrate how this technology can significantly improve healthcare efficiency, decision-making, and patient outcomes. Let’s look at some practical applications and their outcomes.
Case Study 1: Streamlining Clinical Workflows in a Multi-Specialty Hospital
The Challenge: A multi-specialty hospital faced challenges with fragmented patient data and lengthy documentation processes, leading to delays in care delivery.
The Solution: The hospital implemented an AI-powered EMR system equipped with natural language processing (NLP) to automatically transcribe and organize physicians’ notes. The system also used predictive analytics to identify high-risk patients requiring immediate attention.
The Results:
- Reduced time spent on documentation by 35%.
- Improved identification of high-risk patients, reducing emergency readmissions by 20%.
- Enhanced clinician satisfaction due to minimized administrative burden.
Case Study 2: Improving Diagnostic Accuracy in a Regional Clinic
The Challenge: Clinicians at a regional clinic struggled with inconsistent diagnoses due to incomplete patient data and limited access to advanced diagnostic tools.
The Solution: The clinic integrated an AI-powered EMR system that cross-referenced patient symptoms with large medical datasets to suggest possible diagnoses. The system provided real-time alerts for potential drug interactions and flagged abnormal test results.
The Results:
- Increased diagnostic accuracy by 30%.
- Reduced medication errors by 25%.
- Enabled faster treatment planning, cutting patient waiting times by 40%.
Case Study 3: Enhancing Data Interoperability for a Healthcare Network
The Challenge: A large healthcare network had difficulties consolidating patient data from various facilities, leading to incomplete records and delayed treatments.
The Solution: An AI-driven EMR system was implemented to unify data from different sources, ensuring all facilities had access to complete and up-to-date patient records. The system also used machine learning to predict patient admission trends, helping administrators allocate resources effectively.
The Results:
- Achieved 95% data consistency across all facilities.
- Improved resource allocation, reducing patient wait times during peak hours by 15%.
- Increased patient satisfaction scores by 20%.
Case Study 4: Enhancing Preventative Care in a Primary Care Setting
The Challenge: A primary care clinic aimed to focus on preventive care but lacked tools to identify patients at risk of chronic conditions.
The Solution: The clinic adopted an AI-powered EMR system that analyzed patient histories and lifestyle factors to predict the likelihood of chronic diseases like diabetes or hypertension. The system automatically generated personalized care plans and reminders for follow-ups.
The Results:
- Increased early detection of chronic conditions by 40%.
- Enhanced patient adherence to care plans by 25%.
- Reduced long-term healthcare costs by promoting preventative care.
Why These Case Studies Matter
These examples illustrate the transformative power of AI in EMR systems across various healthcare settings. They highlight how this technology can improve efficiency, reduce errors, and enhance patient outcomes when tailored to specific challenges.
Future Outlook: AI in Healthcare and EMR Systems
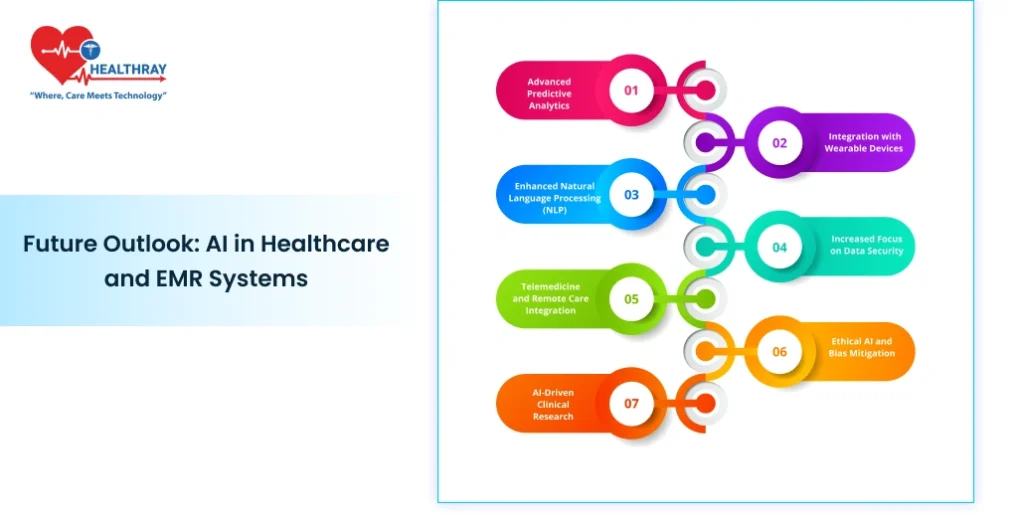
The integration of artificial intelligence (AI) into Electronic Medical Record (EMR) systems is still evolving, with exciting possibilities on the horizon. These advancements promise to reshape how healthcare professionals manage data, interact with patients, and make clinical decisions.
Advanced Predictive Analytics
AI in EMR systems will continue to advance predictive capabilities, enabling healthcare providers to:
- Forecast Disease Trends: Use population health data to predict outbreaks and chronic disease prevalence.
- Personalize Treatment Plans: Offer more precise treatment recommendations based on genetic and lifestyle factors.
- Prevent Hospital Readmissions: Identify at-risk patients and implement proactive interventions.
Integration with Wearable Devices
The future of AI-powered EMR systems includes seamless integration with wearable health devices. This will:
- Allow real-time data collection from devices like smartwatches and fitness trackers.
- Enable continuous monitoring of vital signs for early detection of health issues.
- Provide comprehensive patient profiles that include lifestyle and environmental factors.
Enhanced Natural Language Processing (NLP)
NLP technology will evolve to improve the usability of EMR systems for healthcare providers. Future advancements could:
- Enable better transcription of complex medical terminology.
- Provide multilingual support for global healthcare organizations.
- Simplify interaction with EMR systems through voice commands.
Increased Focus on Data Security
As AI becomes more integrated, ensuring data privacy and security will be paramount. Innovations in this area may include:
- Blockchain technology to create tamper-proof medical records.
- AI-driven algorithms to detect and prevent cyberattacks in real-time.
- Improved compliance with global data protection regulations.
Telemedicine and Remote Care Integration
The rise of telemedicine will push AI-powered EMR systems to support remote care more effectively. This could include:
- AI-driven diagnostic tools for virtual consultations.
- Integration of patient data from remote devices for comprehensive assessments.
- Streamlined workflows for hybrid (in-person and virtual) care models.
Ethical AI and Bias Mitigation
Ethical considerations will take center stage as AI in EMRs becomes more prevalent. Future developments will likely focus on:
- Eliminating bias in AI algorithms to ensure equitable patient care.
- Transparent AI decision-making processes to enhance trust among users.
- Regular audits of AI tools to align with ethical standards.
AI-Driven Clinical Research
AI-powered EMR systems will increasingly support clinical trials and research by:
- Identifying suitable participants through advanced data analysis.
- Accelerating the discovery of new treatment protocols.
- Ensuring accurate and comprehensive data collection.
Why the Future Looks Promising
The ongoing evolution of AI-powered EMR systems will empower healthcare professionals to deliver more accurate, efficient, and patient-centered care. By embracing these advancements, healthcare organizations can stay ahead in a rapidly changing landscape.
Conclusion
AI-powered EMR systems are no longer just tools for record-keeping—they are central to revolutionizing healthcare. These systems streamline administrative tasks, enhance clinical decision-making, and improve patient outcomes. From reducing documentation burdens for clinicians to enable predictive insights for administrators and IT professionals, the benefits are substantial and far-reaching.
However, the road to successful implementation requires careful planning. Organizations must address challenges like data security, interoperability, and staff training to realize the full potential of these systems. The future of healthcare is tied closely to technology, and AI-powered EMRs stand as a vital component of this transformation.
By investing in these systems today, healthcare organizations can position themselves for a future where patient care is smarter, faster, and more personalized. The opportunities are vast, and the time to act is now.