AI has emerged as a transformative force across industries, and healthcare is no exception. In the realm of electronic medical records (EMRs), the integration of AI promises to address some of the most pressing challenges, from improving data accuracy to reducing administrative overload. EMR systems are critical in modern healthcare, serving as the backbone for patient documentation, medical history, and treatment planning. However, inefficiencies, data overload, and manual errors often hinder their effectiveness.
This is where AI steps in to bridge the gaps. From automating routine tasks to offering predictive analytics that assist in clinical decision-making, AI is reshaping how EMRs function. This article dives into the role AI plays in optimizing EMR Softwares, highlighting its benefits, challenges, and future potential for healthcare administrators, software developers, and clinicians.
Benefits of AI Integration in EMR Systems
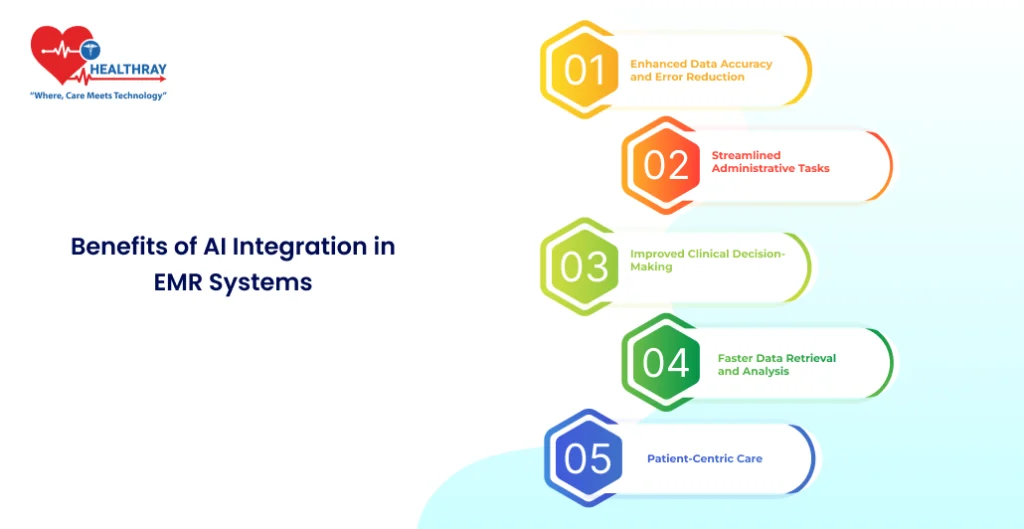
AI integration in EMR systems offers solutions to many of the inefficiencies plaguing traditional systems. By combining computational power with healthcare expertise, AI makes EMR systems smarter and more effective. Here are some of the key benefits:
Enhanced Data Accuracy and Error Reduction
Manual data entry in EMRs is prone to errors, which can have significant consequences for patient care. AI-powered tools can automatically process and organize medical data, reducing the chances of mistakes. For example, natural language processing (NLP) allows clinicians to input notes in plain language, which are then structured by AI into accurate and searchable records.
Streamlined Administrative Tasks
Administrative burdens in healthcare often lead to burnout among clinicians. AI can automate time-consuming tasks like billing, scheduling, and claims processing. This frees up healthcare staff to focus on more meaningful work, such as patient care, while ensuring that processes run efficiently.
Improved Clinical Decision-Making
AI-driven analytics can help clinicians make more informed decisions. Machine learning algorithms analyze patient data, spotting patterns and predicting outcomes that might otherwise go unnoticed. For instance, AI can alert physicians to potential risks, such as early signs of sepsis, or recommend personalized treatment plans based on patient history.
Faster Data Retrieval and Analysis
AI improves how data is accessed and analyzed within EMRs. For example, predictive algorithms can summarize a patient’s medical history and highlight critical information before a consultation. This reduces time spent searching through records and ensures that vital details are not overlooked.
Patient-Centric Care
AI enables EMRs to provide more personalized care recommendations. By analyzing vast datasets, AI can identify trends specific to a patient’s demographics or medical history. This not only improves outcomes but also enhances the overall patient experience.
Challenges in Implementing AI in EMR Systems
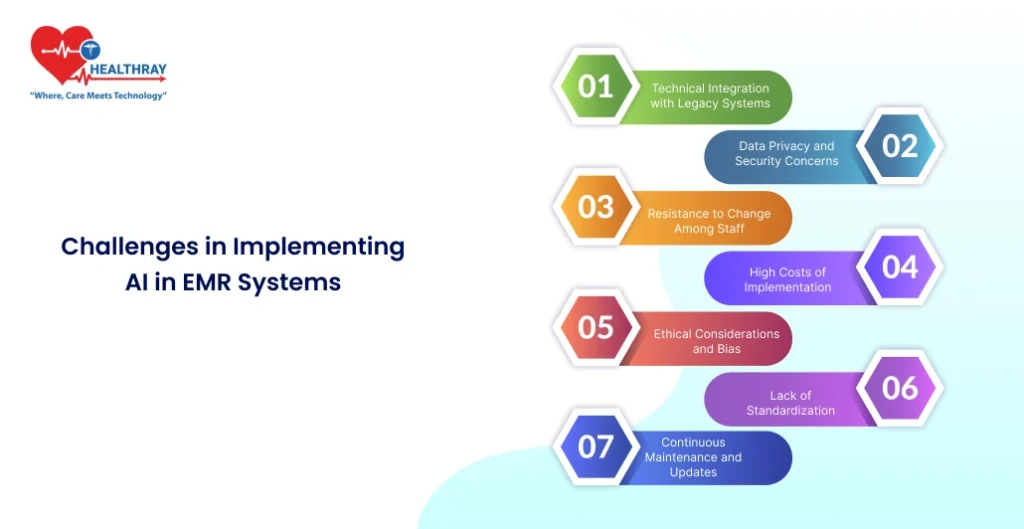
While AI brings significant potential to optimize EMR systems, its implementation is not without challenges. For healthcare organizations looking to adopt AI, understanding these obstacles is crucial for successful integration.
Technical Integration with Legacy Systems
Most healthcare facilities operate on legacy EMR systems that were not built with AI in mind. Integrating AI into these systems requires significant reengineering, including updating software and ensuring compatibility. This process can be time-consuming and costly, especially for institutions with limited IT resources.
Data Privacy and Security Concerns
AI systems require vast amounts of data to function effectively. However, patient information is highly sensitive, and any breaches can lead to serious legal and ethical consequences. Ensuring data security while enabling AI to access and analyze records is a complex balancing act. Strict regulations like HIPAA in the United States add another layer of compliance that organizations must navigate.
Resistance to Change Among Staff
Healthcare providers and administrators often hesitate to adopt new technologies due to concerns about workflow disruptions and the learning curve involved. Staff accustomed to manual processes may view AI as a threat to their roles or fear it will complicate their tasks instead of simplifying them.
High Costs of Implementation
The financial investment required for AI integration can be prohibitive for smaller healthcare facilities. Costs include acquiring AI software, upgrading infrastructure, and training staff. Demonstrating a clear return on investment (ROI) is essential to gain buy-in from stakeholders.
Ethical Considerations and Bias
AI systems are only as good as the data they are trained on. If the training data contains biases, the AI can perpetuate those biases, leading to unfair or inaccurate outcomes. For example, an AI model trained primarily on data from one demographic group might not perform equally well for others, raising concerns about equity in care.
Lack of Standardization
The healthcare industry lacks standard practices for integrating AI into EMR systems. This creates inconsistencies in how AI tools are adopted, making it difficult for organizations to benchmark performance or learn from one another.
Continuous Maintenance and Updates
AI systems are not static. They require regular updates to keep pace with new medical knowledge, regulatory changes, and evolving technology. Ensuring that AI-powered EMRs remain up to date demands ongoing investment in both time and resources.
Case Studies of Successful AI Integration
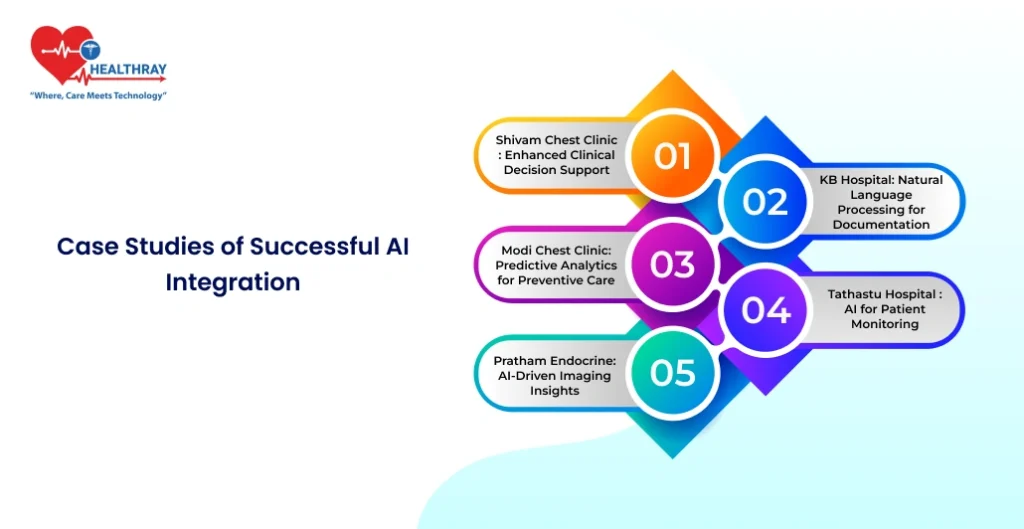
Examining real-world examples of how AI has transformed EMR systems helps highlight its potential and provides actionable insights for others considering similar implementations.
Shivam Chest Clinic : Enhanced Clinical Decision Support
The Shivam Chest Clinic integrated AI into its EMR system to provide real-time clinical decision support. By analyzing patient data and identifying risk factors, their AI-powered system helped physicians detect early warning signs of complications, such as sepsis. This integration reduced adverse events and improved patient outcomes while streamlining workflows for clinicians.
KB Hospital: Natural Language Processing for Documentation
The KB Hospital implemented natural language processing (NLP) technology within its EMR to improve documentation efficiency. Physicians could dictate notes in everyday language, which the AI then structured into organized records. This approach saved time on manual data entry and enhanced the accuracy of medical records, reducing administrative strain.
Modi Chest Clinic: Predictive Analytics for Preventive Care
Modi Chest Clinic utilized AI-driven predictive analytics in its EMR system to identify patients at risk of chronic conditions, such as diabetes and heart disease. The system flagged high-risk individuals and suggested personalized preventive care plans. This not only improved early intervention rates but also reduced long-term healthcare costs.
Tathastu Hospital : AI for Patient Monitoring
Tathastu Hospital integrated AI with its EMR to monitor patient vitals and alert clinicians to potential emergencies. For instance, the AI system analyzed heart rate, blood pressure, and other indicators to detect signs of deterioration. This proactive approach led to faster responses in critical situations, enhancing patient safety.
Pratham Endocrine: AI-Driven Imaging Insights
Pratham Endocrine incorporated AI into its EMR to process imaging data and provide actionable insights to clinicians. AI algorithms helped radiologists identify abnormalities in X-rays and MRIs more efficiently. These insights were seamlessly integrated into the patient’s EMR, allowing for quicker diagnosis and treatment planning.
Key Takeaways from These Case Studies
- Improved Patient Outcomes: AI consistently enhanced the quality of care by providing timely insights and personalized recommendations.
- Streamlined Workflows: Automation of repetitive tasks and efficient data processing reduced administrative burdens on clinicians.
- Cost Efficiency: Early detection and preventive care strategies helped organizations save costs in the long term.
- Scalability: Successful implementations demonstrated that AI systems could scale to meet the needs of large healthcare networks.
Cost Implications and ROI of AI Integration in EMR Systems
Investing in AI-powered EMR systems can seem daunting due to the upfront costs and infrastructure requirements. However, understanding the financial implications and potential return on investment (ROI) can help healthcare organizations make informed decisions.
Initial Costs of Implementation
- Software Acquisition and Licensing: AI solutions often come with significant licensing fees. Custom-built AI tools may require even higher investments compared to off-the-shelf options.
- Infrastructure Upgrades: Many legacy EMR systems require hardware and software upgrades to support AI functionalities, such as cloud computing or advanced processing capabilities.
- Training and Change Management: Educating healthcare staff to effectively use AI-powered tools is critical. Training programs, workshops, and support services add to the initial cost.
Operational Costs
- Maintenance and Support: AI systems need ongoing updates to align with new medical practices, software improvements, and regulatory requirements.
- Data Management Costs: Collecting, cleaning, and maintaining large datasets for AI systems can increase operational expenses.
Return on Investment
Despite the upfront costs, organizations often realize substantial ROI through:
- Increased Efficiency: Automating repetitive tasks such as data entry, billing, and patient scheduling reduces labor costs and minimizes human error.
- Reduced Errors and Better Outcomes: Avoiding medical errors through predictive analytics can significantly lower costs associated with malpractice claims and patient readmissions.
- Shorter Hospital Stays: Proactive alerts and timely interventions enabled by AI can lead to shorter hospital stays, cutting down costs for both patients and providers.
- Improved Revenue Cycle Management: AI streamlines coding and billing processes, reducing claim denials and accelerating reimbursements.
Long-Term Cost Savings
AI-integrated EMRs often pay for themselves over time:
- Preventive Care: Early identification of risks like chronic diseases reduces the need for expensive treatments later.
- Optimized Resource Allocation: Predictive analytics help in better staffing and resource management, preventing underutilization or overuse.
- Enhanced Patient Retention: Satisfied patients are more likely to stay with providers offering advanced, efficient care supported by AI.
Funding and Incentives
Governments and private institutions increasingly offer grants, subsidies, and tax incentives to promote AI adoption in healthcare. Exploring these opportunities can significantly offset initial costs.
Future Trends in AI and EMR Integration
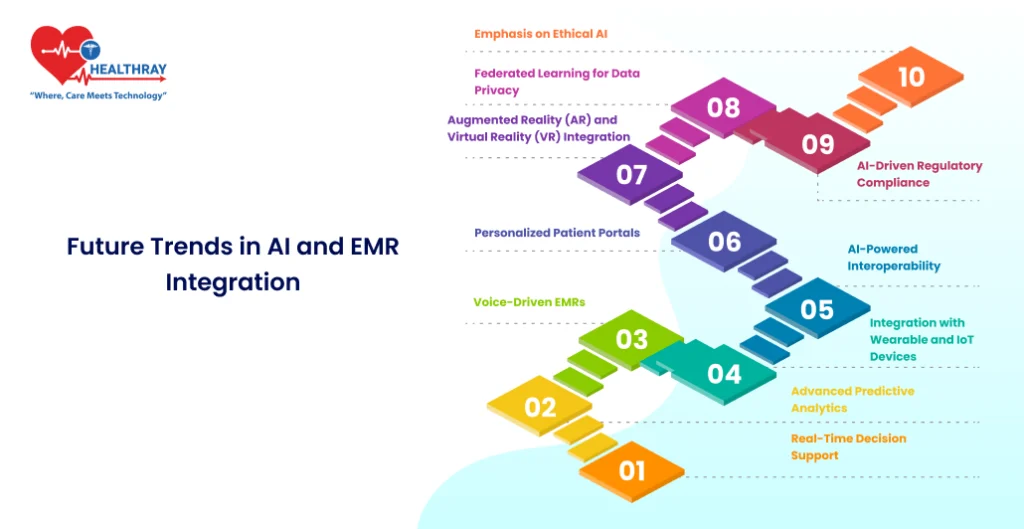
The integration of AI into EMR systems is just the beginning. As technology evolves, new trends are shaping the future of how healthcare organizations manage and utilize electronic medical records. Here’s a look at what’s on the horizon:
Real-Time Decision Support
AI will enable real-time decision support tools embedded directly within EMRs. These systems will continuously analyze patient data and provide clinicians with actionable recommendations during consultations. For example, they may suggest optimal medication doses or flag potential contraindications instantly.
Advanced Predictive Analytics
Predictive analytics will evolve further to provide even deeper insights. AI systems will forecast long-term health outcomes based on historical data, lifestyle factors, and genetic information. This will allow for earlier interventions and highly personalized treatment plans.
Voice-Driven EMRs
Voice recognition technology is set to revolutionize data entry in EMRs. Physicians will interact with AI systems using natural language, reducing reliance on manual inputs. This will save time and improve the accuracy of documentation.
Integration with Wearable and IoT Devices
AI-powered EMRs will seamlessly integrate with wearable devices and Internet of Things (IoT) technologies. Data from devices like smartwatches and remote patient monitoring systems will flow directly into EMRs, offering a comprehensive view of a patient’s health in real time.
AI-Powered Interoperability
Interoperability challenges are a major hurdle for EMR systems. AI will facilitate better data sharing across platforms and institutions, breaking down silos and improving care coordination. This will be particularly valuable in emergency scenarios or for patients receiving care from multiple providers.
Personalized Patient Portals
Future EMRs will offer AI-enhanced patient portals, enabling individuals to access their health data in a user-friendly format. These portals will also provide tailored health advice, reminders, and follow-up care instructions.
Augmented Reality (AR) and Virtual Reality (VR) Integration
AI, combined with AR and VR technologies, will allow EMRs to display 3D models of a patient’s anatomy or treatment plans. This can enhance understanding for both clinicians and patients, particularly in surgical planning or rehabilitation.
Federated Learning for Data Privacy
Federated learning will enable AI systems to train on decentralized data without transferring sensitive information. This approach enhances privacy and compliance with regulations while leveraging data from multiple sources.
AI-Driven Regulatory Compliance
AI will assist in maintaining compliance with healthcare regulations by automating audits and generating reports. It will ensure EMRs stay up-to-date with legal standards, reducing the administrative burden on healthcare organizations.
Emphasis on Ethical AI
As AI becomes more integral to EMRs, there will be a greater focus on ethical AI. Developers and healthcare providers will prioritize transparency, equity, and accountability in AI algorithms to build trust and avoid biases.
Conclusion
AI is transforming electronic medical records (EMRs) from static repositories of information into dynamic tools that improve healthcare delivery. For healthcare administrators, AI-powered EMRs streamline operations, reduce costs, and enhance regulatory compliance. Software developers find opportunities to innovate with scalable solutions that integrate with legacy systems while ensuring security. Clinicians experience reduced burnout through automated tasks and gain actionable insights to improve patient care.The journey to implement AI in the Hospital Management System isn’t without its hurdles. Challenges such as technical integration, data privacy concerns, and cost considerations require careful planning. However, the long-term benefits—improved patient outcomes, operational efficiency, and cost savings—underscore the value of investing in this technology.