How does AI improve security in electronic medical records (EMRs)? This is a question that sparks the interest of anyone involved in healthcare technology. At its core, the security of EMRs ensures sensitive patient data stays protected, preventing breaches that could disrupt care or violate patient privacy. Artificial intelligence (AI) is changing the game in healthcare by providing advanced tools to safeguard these systems.
AI does more than just strengthen firewalls. It anticipates security threats, learns from past incidents, and ensures compliance with ever-changing regulations. In this post, we’ll explore how AI enhances EMR security, dive into real-world applications, and address challenges faced by healthcare professionals, software developers, and administrators.
Understanding AI in EMR Security
Artificial Intelligence in EMR security works like a smart shield, protecting sensitive healthcare data. Unlike traditional methods, AI can analyze vast amounts of information, detect patterns, and anticipate threats before they cause harm. For hospital administrators, EMR software developers, IT professionals, and doctors, this means fewer disruptions and more reliable patient care.
Types of AI Technologies Used in EMR Security
- Machine Learning (ML): Learns from data patterns to identify unusual activity, such as unauthorized access attempts or suspicious data transfers.
- Natural Language Processing (NLP): Helps to interpret and monitor unstructured data, like identifying malicious entries or inconsistencies in medical records.
- Predictive Analytics: Anticipates vulnerabilities and alerts teams about potential security gaps.
- Automated Decision-Making: Reacts to identified threats faster than manual systems, reducing the window of opportunity for hackers.
Why AI is Vital for EMR Security
- Real-Time Monitoring: AI tracks activity 24/7, flagging issues before they escalate.
- Adaptive Learning: It doesn’t just react but evolves. AI adjusts its algorithms to stay ahead of emerging threats.
- Efficient Compliance: Automates compliance checks, ensuring systems meet regulations like HIPAA without constant human oversight.
With these capabilities, AI not only strengthens the security of EMR systems but also allows healthcare professionals to focus on their primary responsibility—patient care.
Enhancing Data Protection with AI
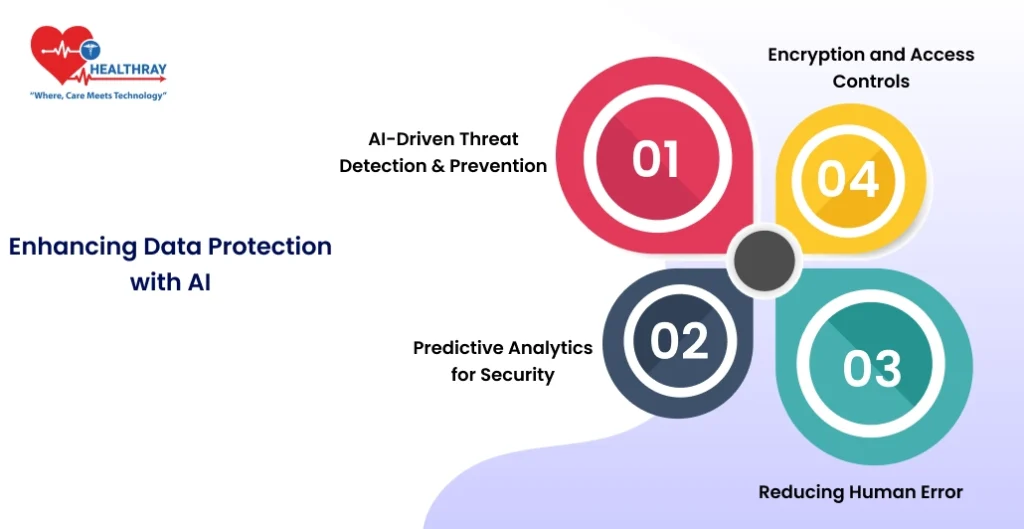
Data protection is the backbone of any EMR system, and AI is raising the bar in safeguarding sensitive patient information. AI-driven tools provide robust defenses against cyber threats by identifying vulnerabilities, detecting breaches in real time, and reducing human error.
AI-Driven Threat Detection and Prevention
AI excels at spotting anomalies in system behavior. For instance:
- Intrusion Detection Systems (IDS): AI-powered IDS can differentiate between normal activities and suspicious actions. This ensures swift identification of unauthorized access attempts.
- Behavioral Analysis: AI tracks user behavior to identify unusual patterns, such as repeated failed login attempts or data access from unrecognized devices.
Predictive Analytics for Security
AI doesn’t just react to threats; it predicts them:
- Identifying Weak Points: AI scans system logs and usage patterns to detect security gaps.
- Anticipating Attacks: It uses historical data and threat intelligence to warn about possible breaches, giving teams a chance to act proactively.
Reducing Human Error
Human mistakes, like misconfigurations or unintentional data exposure, are among the top causes of breaches. AI mitigates this by:
- Automating repetitive tasks to minimize errors.
- Offering intelligent suggestions during workflows, like flagging sensitive data shared without encryption.
Encryption and Access Controls
AI enhances encryption by adapting encryption protocols based on data sensitivity. Additionally, AI can enforce role-based access controls by constantly evaluating whether the right individuals have appropriate access to the data.
By combining these capabilities, AI ensures that Electronic Medical Record Systems remain a step ahead of cybercriminals while maintaining the privacy and security of patient information.
Ensuring Compliance Through AI
Compliance with regulations like HIPAA, GDPR, and other healthcare data protection standards is a constant challenge for hospitals and EMR providers. AI simplifies this by automating compliance processes, identifying potential risks, and ensuring adherence to strict standards without excessive manual effort.
How AI Streamlines Regulatory Compliance
- Automated Auditing and Monitoring:
- AI continuously audits data usage and access logs, ensuring compliance requirements are met in real time.
- It flags any deviations from standard procedures, reducing the risk of non-compliance.
- Data Access Governance:
- AI enforces strict controls over who can access specific patient data. For example, it ensures that only authorized personnel can retrieve sensitive medical records.
- It also tracks and records every interaction with the data, creating a comprehensive audit trail for regulatory purposes.
- Data Anonymization and Privacy Preservation:
- AI can anonymize patient data during analysis, ensuring personal identifiers are protected while allowing research and analytics teams to work with datasets.
- Techniques like differential privacy add an extra layer of security, ensuring that even aggregate data cannot be traced back to individuals.
Meeting Evolving Standards
AI is adaptive, which makes it particularly effective in regulatory environments where rules evolve frequently. By:
- Updating compliance protocols automatically,
- And generating real-time compliance reports, AI reduces the administrative burden on healthcare IT teams and administrators.
Minimizing Fines and Penalties
Non-compliance can lead to heavy fines and loss of reputation. AI mitigates these risks by:
- Identifying gaps before audits.
- Offering suggestions to remediate them quickly.
Through AI, healthcare organizations can ensure their EMR systems not only stay secure but also compliant with the latest regulations, reducing operational and financial risks.
Integrating AI into EMR Systems
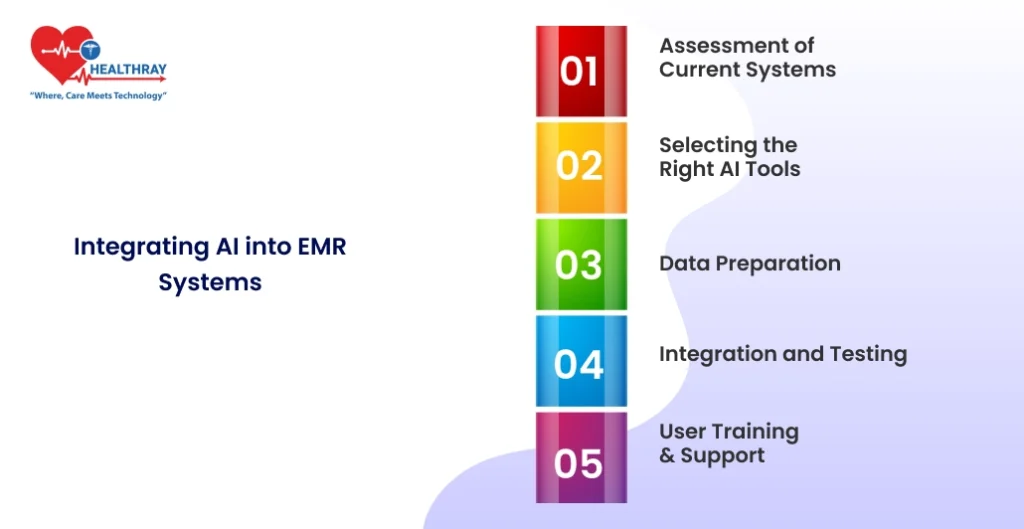
Adding AI to an existing EMR system requires careful planning and execution to ensure seamless integration. While AI brings undeniable benefits, implementing it effectively means addressing compatibility, scalability, and training challenges.
Steps for Successful AI Integration
- Assessment of Current Systems:
- Evaluate the existing EMR infrastructure to identify areas where AI can make the most impact.
- Understand the workflows of administrators, developers, and healthcare professionals to ensure smooth adoption.
- Selecting the Right AI Tools:
- Choose tools that align with your system’s specific needs, such as predictive analytics for threat detection or natural language processing for analyzing records.
- Ensure these tools are scalable and compatible with the EMR system.
- Data Preparation:
- Clean and structure historical data to train AI algorithms. This step is crucial because AI’s effectiveness relies heavily on the quality of data it processes.
- Integration and Testing:
- Embed AI models into the EMR system with minimal disruption to daily operations.
- Conduct rigorous testing in a controlled environment to ensure the AI works as expected and integrates seamlessly with existing workflows.
- User Training and Support:
- Provide training sessions for hospital staff, IT professionals, and doctors to help them understand AI tools and maximize their benefits.
- Set up support teams to address challenges during and after implementation.
Overcoming Common Challenges
- Data Privacy Concerns: Collaborate with AI vendors that prioritize patient data confidentiality and comply with regulations.
- Interoperability Issues: Ensure the AI system can work with other technologies already in use within the healthcare ecosystem.
- Resistance to Change: Communicate the benefits of AI clearly to all stakeholders, focusing on how it enhances security and efficiency.
By following these steps, healthcare organizations can unlock the potential of AI while ensuring a smooth transition and minimal disruption to patient care.
Case Studies: Real-World Applications of AI in EMR Security
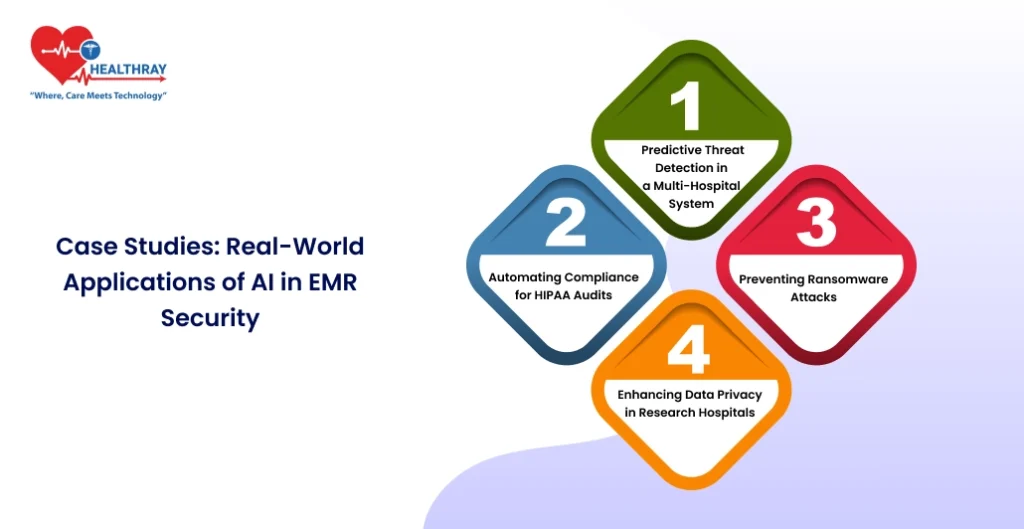
AI is not just a futuristic concept for securing EMRs—it’s already being used in healthcare systems worldwide to safeguard sensitive patient information and streamline operations. Let’s explore a few examples of how organizations are leveraging AI for EMR security.
Predictive Threat Detection in a Multi-Hospital System
A large healthcare network implemented AI-driven predictive analytics to monitor their EMR systems across multiple hospitals. By analyzing access patterns, the AI system flagged unusual login behaviors, such as repeated attempts from unfamiliar IP addresses. These alerts allowed the IT team to prevent potential breaches before they occurred, protecting the records of thousands of patients.
Impact:
- Reduced unauthorized access incidents by 40%.
- Decreased response times for potential threats from hours to minutes.
Automating Compliance for HIPAA Audits
A regional hospital integrated an AI-powered compliance monitoring system into their EMR. The AI tool automatically audited data access logs and flagged areas of non-compliance with HIPAA standards. This not only prepared the hospital for audits but also improved overall data governance.
Impact:
- Cut manual auditing efforts by 70%.
- Improved accuracy in compliance reporting.
Enhancing Data Privacy in Research Hospitals
A research-focused healthcare center used AI to anonymize patient data for analysis without exposing personal identifiers. The system employed differential privacy techniques, allowing researchers to work with the data while keeping patient identities secure.
Impact:
- Enabled large-scale research without compromising privacy.
- Increased collaboration with external researchers due to enhanced data security.
Preventing Ransomware Attacks
An urban hospital network experienced a surge in attempted ransomware attacks targeting their EMR systems. By deploying an AI-based intrusion detection system, the hospital identified and neutralized malware before it could encrypt patient data.
Impact:
- Completely avoided downtime from ransomware.
- Saved the hospital over $500,000 in potential recovery costs.
Future Trends in AI-Driven EMR Security
The future of EMR security looks promising with continuous advancements in AI. Emerging technologies are set to make electronic medical records safer and more efficient, addressing current challenges while preparing for evolving threats.
Federated Learning for Data Privacy
Federated learning allows AI systems to train on decentralized data. Hospitals can benefit from shared insights without transferring sensitive patient data to external servers. This keeps the data local while still improving AI algorithms globally.
- Why It Matters: Prevents breaches associated with data centralization while enabling collaborative learning.
AI and Blockchain Integration
The combination of AI and blockchain offers unmatched security. Blockchain ensures that patient data remains immutable, while AI provides predictive capabilities to monitor and manage threats.
- Potential Use: Real-time tracking of data access with blockchain’s transparency and AI’s alerting mechanisms.
Zero-Trust Security Models
AI will play a significant role in implementing zero-trust security, which assumes no user or device is trustworthy by default. AI systems will continuously verify users and monitor device behavior to ensure every interaction is secure.
- Future Application: Hospitals can deploy dynamic authentication methods, such as biometric checks paired with behavioral monitoring.
Adaptive AI Models
Future AI models will not only detect threats but also self-update based on new patterns and risks. These adaptive models will provide an extra layer of defense against sophisticated cyberattacks.
- Advancement: Automatically adjusts to emerging threats without requiring manual updates.
Natural Language Processing (NLP) for Data Anomaly Detection
NLP advancements will allow AI systems to parse and understand unstructured data better. This means identifying malicious edits or inconsistencies in patient records will become faster and more precise.
- Impact: Improved data integrity checks and quicker detection of unauthorized alterations.
AI for Regulatory Evolution
With healthcare regulations constantly evolving, future AI systems will be designed to adapt to new compliance standards. These systems will notify administrators about upcoming changes and ensure the EMR system aligns with them.
- Advantage: Reduced risks of falling behind regulatory updates.
IoT Security in EMR Systems
With more medical devices connected to EMRs via the Internet of Things (IoT), AI will secure these interactions. It will monitor data flow between devices and EMR systems, ensuring no unauthorized entry points are exploited.
- Example Use: AI could detect compromised IoT devices and isolate them from the network.
Conclusion
AI is transforming the way electronic medical records are secured. From real-time threat detection to automating compliance and integrating with advanced technologies like blockchain, it has become an essential tool for safeguarding sensitive patient data. By adopting AI solutions, hospitals, Hospital Management System developers, and IT professionals can focus on their primary goal—delivering quality healthcare—while ensuring the security of their systems.
As cyber threats grow more sophisticated, AI offers the adaptability and intelligence needed to stay ahead. Whether it’s predictive analytics to anticipate breaches, federated learning for enhanced privacy, or automating compliance for evolving regulations, AI empowers healthcare organizations to protect patient trust and maintain operational integrity.
Investing in AI-driven EMR security isn’t just about prevention—it’s about building a resilient system that evolves with the challenges of tomorrow. The time to act is now.