Artificial Intelligence (AI) is revolutionizing the way healthcare operates, and its integration into Electronic Health Records (EHR) is a significant leap forward. For healthcare professionals and doctors, managing vast amounts of patient data has always been a time-consuming challenge. EHR systems alleviated some of that burden, but they still require extensive manual input and lack intelligent analysis capabilities. AI bridges this gap by transforming EHR systems into smarter, more efficient tools.
This integration goes beyond automating routine tasks. AI can assist in predicting patient outcomes, identifying at-risk individuals, and streamlining workflows. But, like every innovation, it comes with challenges. High implementation costs, data privacy concerns, and resistance to change are just a few hurdles to address.
In this article, we’ll explore the benefits and challenges of integrating AI into EHR Software. Along the way, we’ll uncover real-world examples, share best practices, and provide actionable insights for healthcare professionals, developers, and doctors alike.
What is AI in EHR Integration?
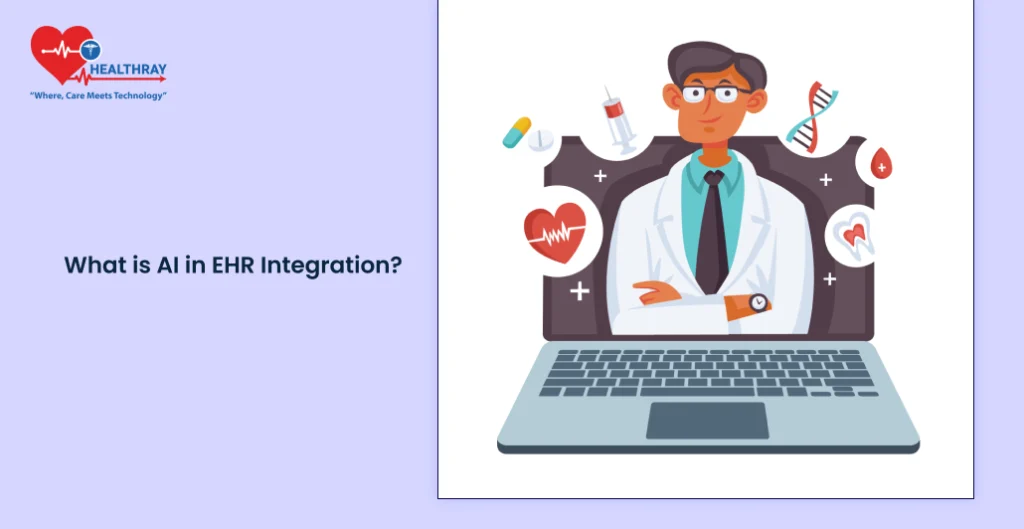
AI in Electronic Health Record (EHR) integration refers to the use of advanced algorithms and machine learning models to enhance the functionality, usability, and efficiency of EHR systems. Traditional EHR platforms have primarily been digital repositories for patient data, often requiring significant manual input and offering limited analytical insights. AI shifts this paradigm by making EHRs more intelligent and user-friendly.
A Brief History of EHR and AI Evolution
EHR systems were introduced to digitize patient records, replacing paper charts and improving accessibility. Over time, as healthcare demands grew, these systems were pushed to incorporate more advanced features like data-sharing capabilities and clinical decision support. The integration of AI represents the next step in this evolution. AI not only automates repetitive tasks but also analyzes complex datasets to provide actionable insights.
Key AI Tools in EHR Systems
- Predictive Analytics: These tools analyze patient history and trends to predict potential health outcomes or risks. For instance, they can flag patients likely to develop chronic conditions.
- Natural Language Processing (NLP): NLP tools can convert unstructured data, such as physician notes, into structured formats for easier analysis and retrieval.
- Virtual Assistants: These assist healthcare professionals by automating scheduling, reminders, and even initial diagnostic processes.
- Image and Data Analysis: AI can process medical images or large datasets, aiding in faster and more accurate diagnoses.
Why This Matters
For healthcare professionals, the integration of AI into EHR systems means less time spent on administrative tasks and more focus on patient care. Doctors benefit from improved clinical decision support, while developers gain opportunities to innovate smarter, more connected systems.
Benefits of AI in EHR for Stakeholders
AI integration in EHR systems delivers significant advantages for healthcare professionals, doctors, and software developers. These benefits not only streamline workflows but also improve patient outcomes and open up opportunities for innovation.
For Healthcare Professionals
- Reduced Administrative Burden: AI automates repetitive tasks like data entry, appointment scheduling, and documentation, allowing healthcare professionals to focus on patient care.
- Streamlined Workflows: With features like automated reminders and predictive scheduling, processes become more efficient, saving time and reducing errors.
For Doctors
- Enhanced Clinical Decision Support: AI tools analyze patient data to identify trends, flag potential risks, and suggest treatment options. For instance, a system might alert a doctor to early signs of sepsis based on vital signs and lab results.
- Improved Diagnostic Accuracy: Machine learning models can process large datasets, including medical images, to help doctors diagnose conditions more quickly and accurately.
- Personalized Care Plans: AI enables tailored treatment strategies by analyzing individual patient histories and outcomes.
For EHR Software Developers
- Innovation Opportunities: Developers can design smarter, more efficient systems that offer predictive analytics, voice recognition, and real-time data processing.
- Market Competitiveness: Integrating AI into Electronic Health Record platforms provides a competitive edge, as healthcare organizations increasingly seek intelligent systems.
- Scalability and Customization: AI-powered systems can adapt to the unique needs of various healthcare facilities, from small clinics to large hospitals.
Real Impact
For stakeholders across the board, the benefits of AI in EHR integration extend far beyond convenience. These tools can save lives by flagging critical issues earlier, reduce burnout by automating mundane tasks, and push the boundaries of what healthcare technology can achieve.
Challenges of AI in EHR Integration
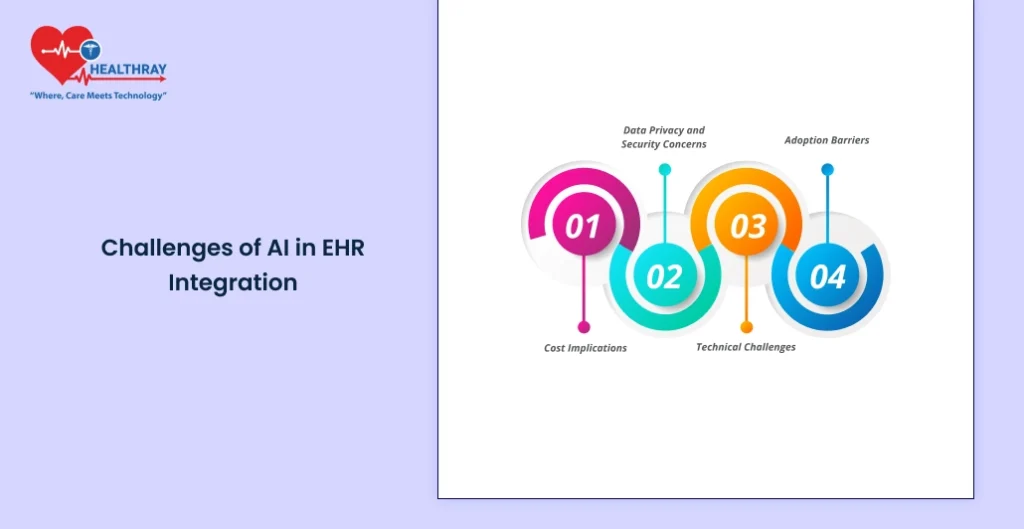
While the benefits of integrating AI into EHR systems are clear, it’s not without significant challenges. From high costs to technical and ethical issues, these hurdles can slow adoption and create barriers for stakeholders.
Cost Implications
- High Initial Investment: Implementing AI in EHR systems requires significant upfront costs for software development, hardware upgrades, and staff training.
- Ongoing Maintenance: AI systems need regular updates to remain effective, which adds to operational expenses.
- Resource Allocation: Smaller clinics or healthcare facilities might struggle to allocate funds for these technologies.
Data Privacy and Security Concerns
- Sensitive Information: EHR systems store extensive patient data, making them prime targets for cyberattacks.
- Compliance Issues: Ensuring compliance with laws like HIPAA and GDPR adds complexity to AI integration.
- Trust Deficit: Patients and professionals may worry about how AI systems handle sensitive data, slowing acceptance.
Technical Challenges
- Integration with Legacy Systems: Many healthcare providers still rely on older systems that are incompatible with modern AI solutions.
- Data Standardization: The lack of consistent data formats makes it harder for AI systems to process and analyze information effectively.
- Accuracy and Reliability: AI models require large volumes of quality data for training. Inconsistent or incomplete datasets can lead to inaccurate predictions.
Adoption Barriers
- Resistance to Change: Some healthcare professionals may be hesitant to trust AI systems, especially if they perceive them as overly complex or intrusive.
- Training Requirements: Staff need extensive training to use AI-powered EHR systems effectively, which can disrupt workflows during implementation.
- Usability Issues: Poorly designed interfaces can create frustration and reduce the perceived value of AI tools.
Addressing these challenges requires collaboration between healthcare professionals, developers, and policymakers. Overcoming these barriers is essential for the successful integration of AI into EHR systems.
Real-World Examples of AI in EHR
The integration of AI into EHR systems is already yielding tangible benefits in healthcare settings. These real-world examples demonstrate how AI is improving efficiency, enhancing patient care, and paving the way for future innovations.
Predictive Analytics for Patient Care
Several hospitals have implemented AI-driven predictive analytics tools to identify high-risk patients. For instance:
- Sepsis Prediction: AI models analyze patient vitals, lab results, and historical data to detect early signs of sepsis. This allows healthcare teams to intervene sooner, reducing mortality rates.
- Chronic Disease Management: AI in EHR systems flags patients at risk of diabetes or hypertension based on lifestyle factors and medical history. Tailored care plans can then be implemented.
Natural Language Processing (NLP) in Documentation
Healthcare providers are using NLP tools to simplify clinical documentation. These tools extract relevant data from unstructured notes, such as:
- Converting doctor-patient conversations into structured EHR entries.
- Summarizing lengthy medical histories for quick review.
Virtual Health Assistants
AI-powered virtual assistants are being integrated into EHR platforms to automate routine tasks, including:
- Appointment scheduling and reminders.
- Patient follow-up messaging.
- Answering common queries, reducing the burden on administrative staff.
Imaging and Diagnostics Integration
AI in EHR systems supports radiologists by:
- Analyzing medical images for signs of cancer, fractures, or other conditions.
- Integrating imaging results directly into the patient’s EHR, offering a seamless view for doctors.
Medication Management
Pharmacies and hospitals use AI-integrated EHR tools to:
- Detect potential drug interactions based on patient prescriptions.
- Monitor medication adherence through smart pill dispensers linked to the EHR system.
Best Practices for Implementing AI in EHR
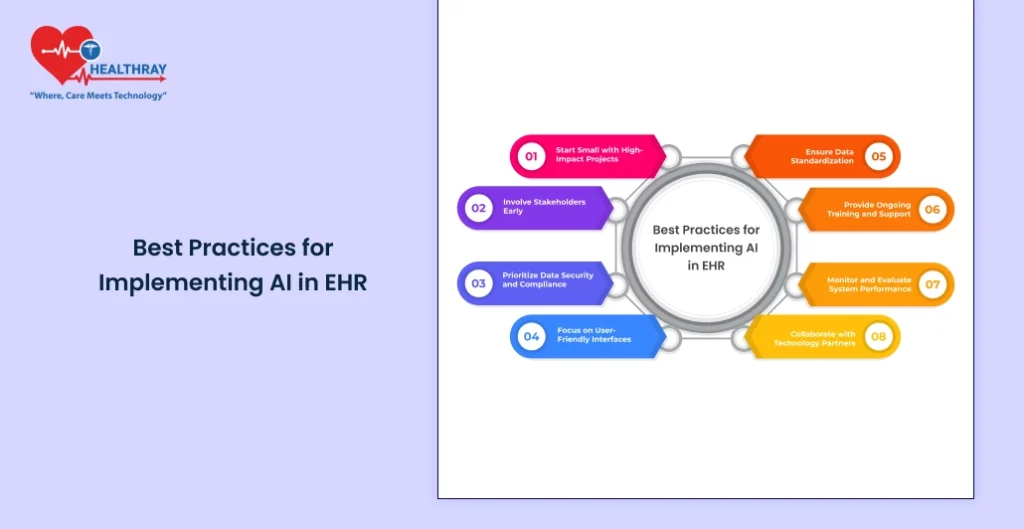
Successfully integrating AI into EHR systems requires a strategic approach. By following best practices, healthcare providers and developers can maximize the benefits of AI while addressing common challenges.
Start Small with High-Impact Projects
- Focus on specific areas where AI can bring immediate improvements, such as automating administrative tasks or enhancing clinical decision-making.
- Pilot projects in a controlled environment to identify potential issues before scaling.
Involve Stakeholders Early
- Engage healthcare professionals, doctors, and administrative staff in the decision-making process. Their insights can shape the system to meet practical needs.
- Conduct regular feedback sessions to refine AI tools during implementation.
Prioritize Data Security and Compliance
- Ensure the system complies with privacy regulations like HIPAA and GDPR.
- Use encryption and advanced cybersecurity measures to protect sensitive patient data.
- Establish clear policies for data access and usage to build trust among users.
Focus on User-Friendly Interfaces
- Design intuitive, easy-to-use interfaces that reduce the learning curve for staff.
- Incorporate training modules and support resources directly into the EHR platform.
- Regularly update the system based on user feedback to enhance functionality.
Ensure Data Standardization
- Standardize patient data formats across the organization to improve compatibility with AI tools.
- Use data-cleaning techniques to eliminate errors and inconsistencies in records.
Provide Ongoing Training and Support
- Train staff thoroughly on the features and functionalities of AI-integrated EHR systems.
- Offer continued support through help desks, tutorials, and on-site sessions.
- Highlight real-world use cases to demonstrate the practical benefits of AI tools.
Monitor and Evaluate System Performance
- Regularly assess the impact of AI on workflows, patient outcomes, and staff satisfaction.
- Use analytics to identify areas where AI tools can be optimized.
- Adapt the system as healthcare needs and technological advancements evolve.
Collaborate with Technology Partners
- Work with experienced AI and EHR developers to ensure seamless integration.
- Choose vendors who prioritize transparency and customization to meet your specific needs.
Following these best practices can help healthcare organizations harness the full potential of AI in EHR systems while avoiding common pitfalls. A thoughtful implementation strategy ensures that both staff and patients benefit from this cutting-edge technology.
Conclusion
The integration of AI into EHR systems has the potential to transform healthcare. By automating repetitive tasks, enhancing decision-making, and personalizing patient care, AI offers benefits that touch every stakeholder, from doctors to developers. However, this progress comes with challenges, including high costs, data security concerns, and usability issues.
To make the most of AI in the Hospital Management System, it’s essential to adopt a strategic approach. Starting small, engaging stakeholders, and focusing on data security and user-friendly design can pave the way for successful implementation. Real-world examples show that the effort is worth it, as AI tools help save lives, reduce administrative burdens, and improve healthcare outcomes.
As the healthcare industry continues to evolve, AI-integrated EHR systems will play an increasingly central role. By addressing challenges thoughtfully and embracing best practices, healthcare providers and developers can unlock the full potential of this groundbreaking technology.