AI is revolutionizing how hospitals manage Electronic Health Records (EHR), bringing a new level of efficiency and insight to patient care. By automating mundane tasks, providing real-time analytics, and enhancing decision-making, AI-powered EHR systems are making life easier for healthcare providers and improving patient outcomes.
This article will explore how hospitals are integrating AI into EHR Softwares, the tangible benefits it delivers, and the challenges that come with it. We’ll also look at real-world examples and emerging trends shaping the future of AI in healthcare.
The Benefits of AI Integration in EHR Systems
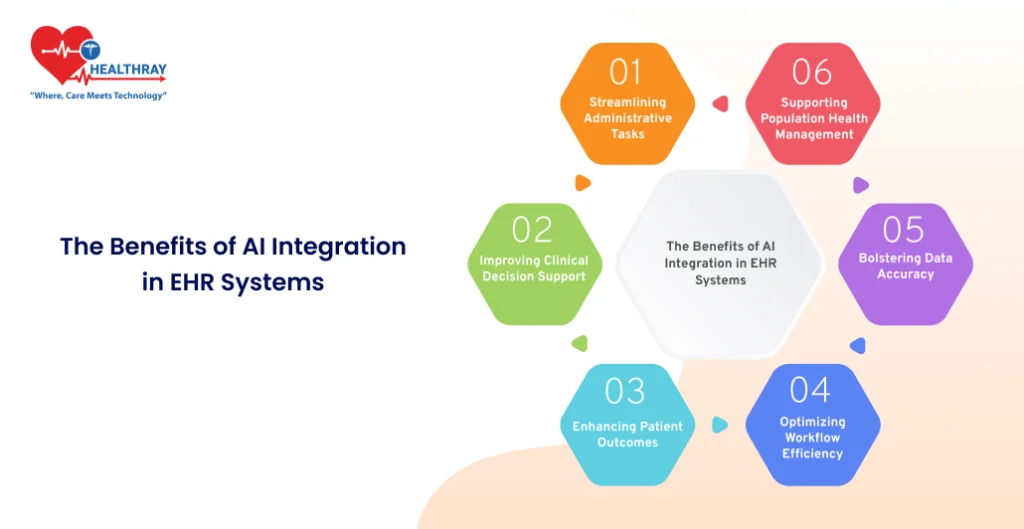
AI is transforming Electronic Health Records (EHR) from static repositories into dynamic tools that actively improve hospital operations and patient care. Here’s how AI is enhancing EHR systems:
Streamlining Administrative Tasks
Hospital staff often spend significant time on documentation, data entry, and chart management. AI-powered tools automate these processes, reducing administrative burdens. For example, natural language processing (NLP) can transcribe clinical notes in real-time, allowing physicians to focus more on patient interaction rather than paperwork.
Improving Clinical Decision Support
AI integrates seamlessly with EHR systems to analyze patient data and provide actionable insights. Predictive analytics can alert clinicians to potential complications before they arise, such as detecting early signs of sepsis or adverse drug interactions. These tools serve as an additional layer of support, improving decision-making and reducing human error.
Enhancing Patient Outcomes
By leveraging AI, hospitals can identify at-risk patients and personalize care plans based on historical data and real-time metrics. This tailored approach increases treatment effectiveness and leads to better recovery rates. AI-driven insights also help in reducing hospital readmission rates by proactively addressing patients’ health needs post-discharge.
Optimizing Workflow Efficiency
AI algorithms help prioritize tasks and streamline scheduling, ensuring resources are allocated efficiently. For instance, machine learning models can predict peak admission times, enabling better staffing and resource planning. These improvements translate to shorter wait times and more efficient patient care.
Bolstering Data Accuracy
Errors in EHR data can have serious consequences for patient care. AI tools validate and correct entries in real-time, ensuring the integrity of records. With enhanced accuracy, healthcare professionals can rely on EHR data with greater confidence.
Supporting Population Health Management
AI analyzes large datasets to identify patterns and trends in patient populations. Hospitals can use these insights to address public health challenges, such as identifying outbreaks or improving care for chronic conditions. This capability allows for proactive measures and a more strategic approach to healthcare delivery.
Implementation Strategies for AI in EHR Systems
Adopting AI in EHR systems requires thoughtful planning and execution to ensure successful integration. Hospitals must address technical, operational, and human factors to maximize AI’s potential. Here are key strategies for effective implementation:
Assess Existing Infrastructure
Before implementing AI, hospitals should evaluate their current EHR systems and IT infrastructure. Compatibility is crucial, as AI solutions must integrate seamlessly with existing platforms. This assessment also identifies gaps that need addressing, such as outdated software or inadequate data storage.
Involve Multidisciplinary Teams
Successful integration requires collaboration between hospital administrators, IT professionals, clinicians, and AI vendors. Each group brings unique perspectives that ensure the solution meets technical and clinical requirements while remaining user-friendly.
Prioritize Data Quality
AI algorithms rely on high-quality, structured data to function effectively. Hospitals must clean and standardize their data to avoid errors and biases. Regular audits and employing tools that validate data integrity are essential steps.
Invest in Staff Training
Introducing AI into EHR systems will only succeed if staff members feel confident using the new tools. Training programs should focus on both the technical and practical aspects of AI-powered features. Hands-on workshops and ongoing support can ease the transition.
Address Security and Compliance
AI in EHR systems involves handling sensitive patient data, making cybersecurity a top priority. Hospitals should implement robust encryption protocols and adhere to regulations like HIPAA. Conducting regular security assessments helps protect against potential breaches.
Start Small with Pilot Programs
Instead of rolling out AI across all departments at once, hospitals can begin with pilot programs in specific areas like radiology or patient scheduling. Pilot programs allow for testing, gathering feedback, and fine-tuning the system before broader implementation.
Focus on Scalability
As hospitals grow and patient needs evolve, their AI solutions must scale accordingly. Choosing flexible systems that can adapt to future demands ensures long-term value from the investment.
Monitor and Evaluate
Post-implementation, hospitals should continuously monitor AI’s impact on both operational efficiency and patient outcomes. Regular evaluations
Challenges and Considerations in AI Integration for EHR Systems
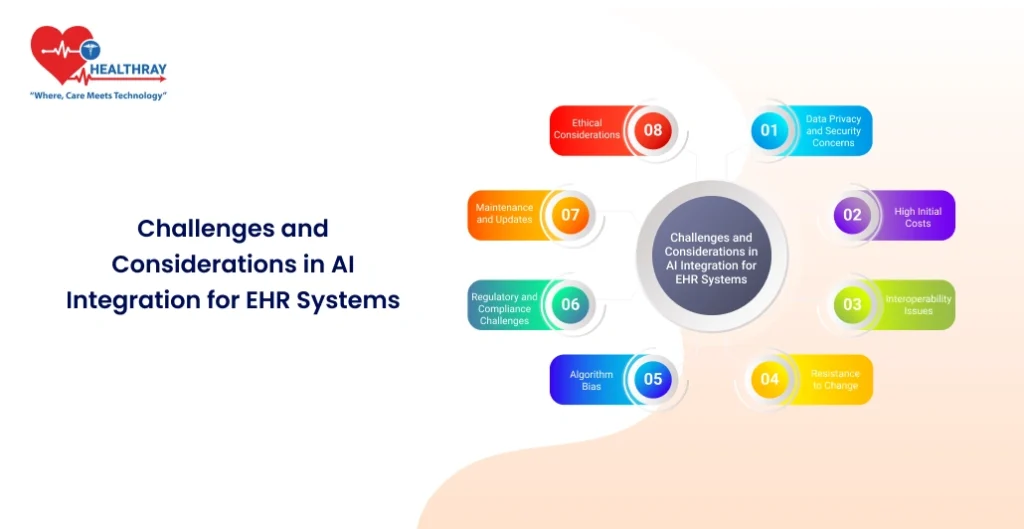
While AI offers transformative potential for EHR systems, its implementation comes with challenges that hospitals must address. Recognizing and preparing for these obstacles ensures smoother integration and maximizes benefits. Here are some key considerations:
Data Privacy and Security Concerns
EHR systems handle highly sensitive patient information. Incorporating AI increases the risk of data breaches and unauthorized access. Hospitals must invest in advanced cybersecurity measures, such as multi-factor authentication, encryption, and regular vulnerability assessments, to safeguard data.
High Initial Costs
AI systems require significant upfront investment in software, hardware, and training. For smaller hospitals with limited budgets, this can pose a barrier. Careful cost-benefit analysis and phased implementation can help manage financial challenges.
Interoperability Issues
EHR systems often operate on different platforms, making seamless integration of AI challenging. Lack of standardization across systems can lead to data silos and reduced effectiveness. Collaboration with vendors to ensure compatibility and the use of open standards can mitigate this issue.
Resistance to Change
Staff may be hesitant to adopt AI due to fears of job displacement or lack of familiarity with the technology. Open communication, emphasizing AI as a support tool rather than a replacement, and robust training programs can help alleviate these concerns.
Algorithm Bias
AI models can inadvertently perpetuate biases present in historical healthcare data. For instance, underrepresented patient populations might receive less accurate predictions or care recommendations. Regular audits and diverse data inputs are essential to minimize bias.
Regulatory and Compliance Challenges
Healthcare AI must comply with stringent regulations like HIPAA in the U.S. or GDPR in Europe. Navigating these frameworks can be complex and may delay implementation. Hospitals must work with legal experts to ensure compliance without compromising functionality.
Maintenance and Updates
AI systems require ongoing updates to stay effective and secure. This includes recalibrating algorithms, patching vulnerabilities, and adapting to changing healthcare protocols. Allocating resources for long-term maintenance is critical.
Ethical Considerations
Relying on AI for critical healthcare decisions raises ethical questions. Transparency in how AI algorithms work and accountability for errors must be established to build trust among patients and clinicians.
Future Trends in AI and EHR Systems
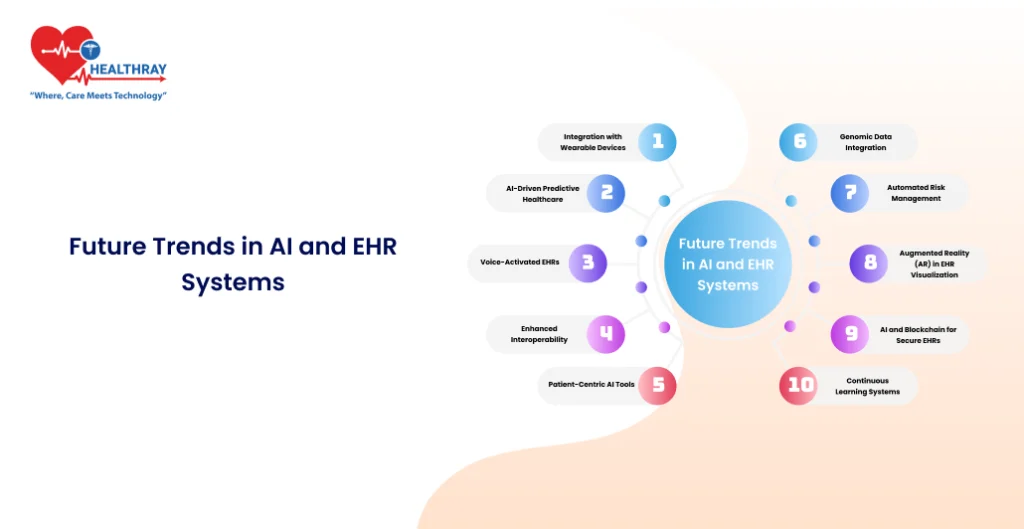
The evolution of AI technology promises even greater advancements in how Electronic Health Record (EHR) systems function. Here’s a look at emerging trends that will shape the future of AI in healthcare:
Integration with Wearable Devices
AI-powered EHR systems will increasingly integrate data from wearable health devices, such as fitness trackers and smartwatches. This real-time data provides clinicians with a more comprehensive view of a patient’s health, aiding in proactive care and personalized treatment plans.
AI-Driven Predictive Healthcare
Future EHR systems will use advanced AI algorithms to predict patient outcomes with even greater accuracy. For example, AI may flag potential health issues like heart attacks or diabetes years in advance, based on historical data and lifestyle patterns.
Voice-Activated EHRs
Voice-enabled AI assistants will become a common feature in EHR systems. These assistants will allow clinicians to update records, retrieve patient information, and receive alerts without typing, making data entry faster and more intuitive.
Enhanced Interoperability
AI will play a pivotal role in breaking down silos between disparate healthcare systems. By facilitating seamless data sharing and standardization, AI will ensure that patient records are accessible and consistent across institutions, improving care coordination.
Patient-Centric AI Tools
The future will see more AI tools designed to empower patients. For instance, AI chatbots integrated into EHR portals will help patients understand their medical records, manage appointments, and receive tailored health advice, fostering better engagement.
Genomic Data Integration
With advances in genomics, AI-driven EHR systems will incorporate genetic information into patient records. This will enable more precise diagnoses and personalized treatment strategies, particularly in fields like oncology and rare disease management.
Automated Risk Management
AI will enhance risk management by continuously monitoring EHR data for anomalies that suggest compliance issues, billing fraud, or data breaches. These systems will offer real-time alerts, allowing hospitals to mitigate risks promptly.
Augmented Reality (AR) in EHR Visualization
Augmented Reality combined with AI will transform how clinicians view EHR data. For example, AR tools might display patient information in a 3D format during surgery or provide interactive visuals for complex case discussions.
AI and Blockchain for Secure EHRs
The convergence of AI with blockchain technology will redefine EHR security. Blockchain ensures data integrity and transparency, while AI analyzes the blockchain for potential security threats, creating a robust defense against cyberattacks.
Continuous Learning Systems
Future AI in EHRs will be equipped with machine learning models that continually adapt based on new data. These self-improving systems will ensure that recommendations and analytics become increasingly accurate and relevant over time.
Conclusion
AI is revolutionizing Electronic Health Records (EHR) systems, offering hospitals a powerful tool to enhance efficiency, improve decision-making, and deliver better patient outcomes. From streamlining administrative tasks to enabling predictive healthcare and personalized treatment plans, the impact of AI is undeniable.
However, adopting AI comes with challenges, including high implementation costs, privacy concerns, and the need for staff training. Addressing these barriers with thoughtful strategies ensures successful integration and long-term benefits. Real-world examples, such as those from Mayo Clinic and Geisinger Health System, highlight the transformative potential of AI when applied effectively.
Looking ahead, AI’s role in Hospital Management Systems will only expand, driven by trends like wearable device integration, patient-centric tools, and genomic data analysis. Hospitals that embrace these advancements will be better equipped to meet the evolving demands of healthcare.
As AI continues to advance, it’s clear that its partnership with EHR systems will be at the forefront of creating a more efficient, patient-centered healthcare ecosystem.