Electronic Health Records (EHRs) are the backbone of modern healthcare. They store patient information digitally, making it easier to access, share, and analyze data. But as the demands on healthcare systems grow, traditional EHRs struggle to keep up. That’s where Artificial Intelligence (AI) steps in, promising a transformation in how healthcare data is managed and used.
AI isn’t just making EHRs faster. It’s helping healthcare professionals make better decisions, reducing administrative burdens, and paving the way for personalized care. This article will explore the different types of EHR Software and how AI is driving innovation, helping stakeholders—from hospital administrators to AI tech companies—understand the possibilities and challenges.
Are you ready to see how EHRs and AI are reshaping healthcare? Let’s dive in.
Let me know if you like this introduction or want any changes before I move on to the first section: Types of Electronic Health Record Systems.
Types of Electronic Health Record Systems
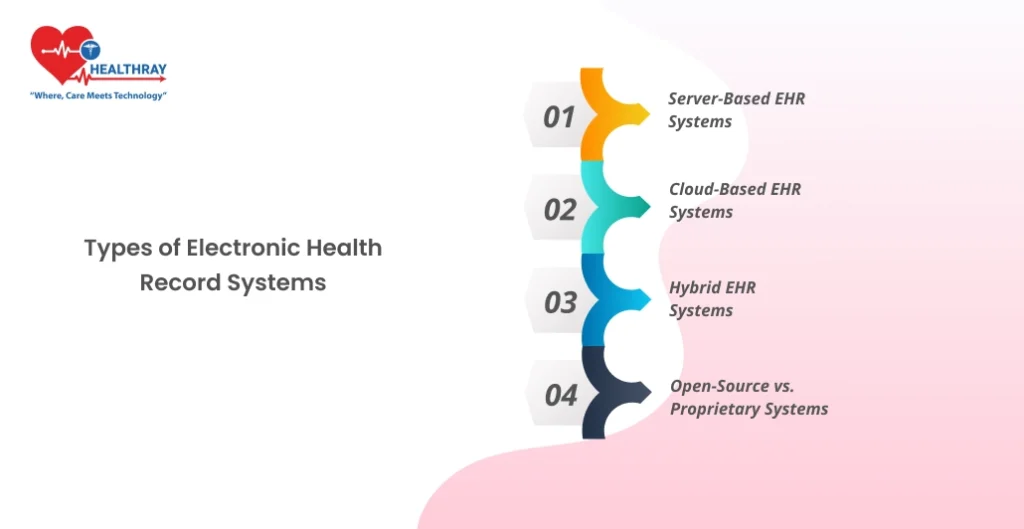
Electronic Health Records come in different forms, each designed to meet the varying needs of healthcare organizations. Let’s break down the main types:
Server-Based EHR Systems
These systems use actual servers inside the medical facility to store data. Because the data is stored locally, they provide a high degree of control and security. Nevertheless, they frequently have drawbacks, such as high initial expenses and the requirement for internal IT teams to oversee and maintain them. Larger hospitals or organizations with specialized infrastructure resources are more likely to use these systems.
Cloud-Based EHR Systems
Cloud-based systems are hosted on external servers and accessed via the internet. They are gaining popularity due to their flexibility and cost-effectiveness. With no need for expensive hardware, smaller clinics and hospitals can adopt them easily. These systems are also scalable, making them suitable for facilities looking to expand.
Hybrid EHR Systems
A hybrid approach combines elements of server-based and cloud-based systems. These systems provide flexibility by allowing sensitive data to be stored on local servers while enabling less critical data to be accessed via the cloud. They balance security with convenience but may require higher upfront investments.
Open-Source vs. Proprietary Systems
- Open-Source EHRs allow healthcare providers to customize the system according to their specific needs. They are often less expensive and community-driven but require technical expertise to implement and maintain.
- Proprietary Systems are developed and maintained by specific vendors. They usually come with better support and regular updates but can be more restrictive and costly.
AI Integration in EHRs
Artificial Intelligence is revolutionizing how healthcare professionals interact with Electronic Health Records. By automating processes and providing deeper insights, AI is making EHR systems smarter and more efficient. Here’s how AI is reshaping EHRs:
Enhancing Data Accuracy and Accessibility
AI-driven tools can identify errors in patient records and suggest corrections, ensuring data integrity. With advanced algorithms, healthcare providers can quickly search and retrieve information, reducing the time spent navigating complex systems.
Predictive Analytics for Patient Care
EHRs can now examine patient data for trends and patterns thanks to AI. Predictive models can evaluate a patient’s risk of developing long-term illnesses like diabetes or heart disease, for instance. This aids physicians in implementing preventative measures and creating individualized treatment plans.
Automating Administrative Tasks
Administrative duties, like coding for insurance claims or updating patient records, are a significant drain on time. AI-powered automation can handle these repetitive tasks, allowing healthcare professionals to focus more on patient care.
Streamlining Clinical Decision Support
AI algorithms can provide real-time suggestions based on patient data, improving clinical decision-making. For instance, they can flag potential drug interactions, recommend treatment options, or alert doctors to abnormal lab results.
Case Studies: Successful AI-EHR Integration
Several healthcare systems have implemented AI-enhanced EHRs with impressive results. Hospitals report reduced errors, faster diagnosis times, and improved patient outcomes. These real-world examples show the tangible benefits of merging AI with healthcare data systems.
Challenges in AI Integration
While the benefits are significant, there are hurdles to overcome, such as:
- Ensuring data privacy and security.
- Integrating AI tools with legacy systems.
- Training staff to use new technologies effectively.
AI isn’t just a buzzword; it’s becoming a critical tool for healthcare providers looking to optimize their operations and deliver better care.
Implementation Challenges and Solutions
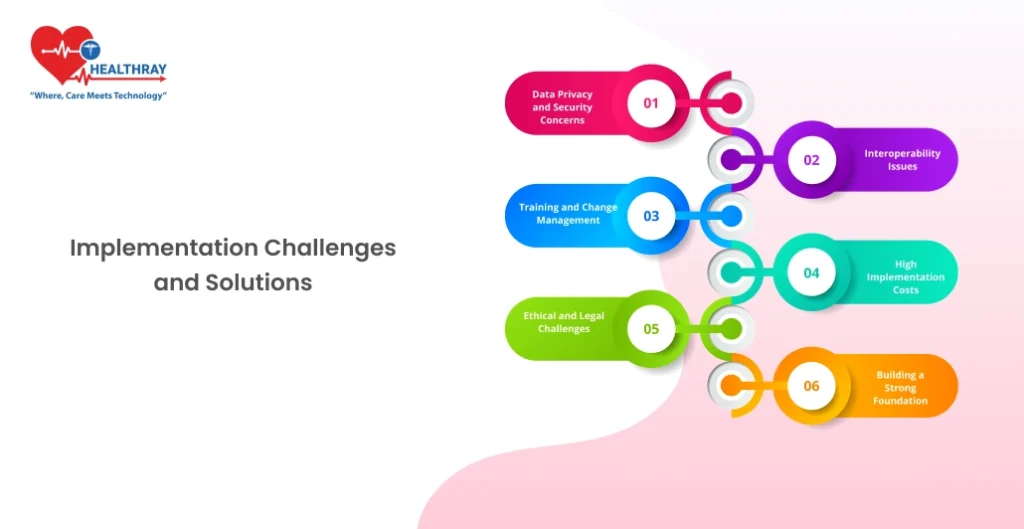
Integrating Artificial Intelligence into Electronic Health Records (EHRs) is no small feat. Despite its potential to revolutionize healthcare, the process is riddled with challenges. Here’s a closer look at the obstacles and practical ways to overcome them.
Data Privacy and Security Concerns
Protecting data privacy is crucial when it comes to sensitive patient information. The need for AI systems to access large datasets raises questions regarding their compliance with laws like GDPR and HIPAA. Significant risks are also posed by cybersecurity threats.
Solution:
- Adopt robust encryption methods to protect data.
- Use anonymized data for AI training to reduce risks.
- Regularly audit systems for vulnerabilities and ensure compliance with data protection laws.
Interoperability Issues
Many healthcare facilities use legacy systems that don’t easily integrate with modern AI tools. Fragmented data across different systems can hinder seamless AI implementation.
Solution:
- Invest in interoperable platforms that support standard data formats like HL7 or FHIR.
- Employ middleware solutions to bridge gaps between old and new systems.
Training and Change Management
AI systems can intimidate healthcare workers unfamiliar with the technology. Resistance to change and lack of adequate training can slow down adoption.
Solution:
- Provide hands-on training sessions tailored to different roles within the organization.
- Highlight real-life success stories to demonstrate the value of AI in simplifying workflows.
- Create a feedback loop to address user concerns promptly.
High Implementation Costs
The upfront costs of AI tools, infrastructure upgrades, and staff training can be prohibitive for smaller facilities.
Solution:
- Start with scalable AI solutions that allow for incremental investments.
- Leverage government grants, partnerships, or collaborations with AI tech companies.
- Focus on use cases with high ROI, such as automated billing or predictive analytics for critical care.
Ethical and Legal Challenges
The use of AI in decision-making can introduce ethical dilemmas, such as bias in algorithms or accountability for AI-driven errors.
Solution:
- Regularly audit AI models to identify and eliminate biases.
- Clearly define roles and responsibilities for AI-driven decisions within the healthcare team.
- Stay informed about evolving legal frameworks governing AI use in healthcare.
Building a Strong Foundation
To successfully implement AI-enhanced EHRs, organizations must:
- Start with pilot programs to test and refine solutions.
- Involve stakeholders across departments to ensure alignment.
- Continuously evaluate performance and adapt to new challenges.
Overcoming these hurdles isn’t easy, but the rewards—streamlined workflows, reduced errors, and better patient care—make the effort worthwhile.
Future Trends in AI and EHRs
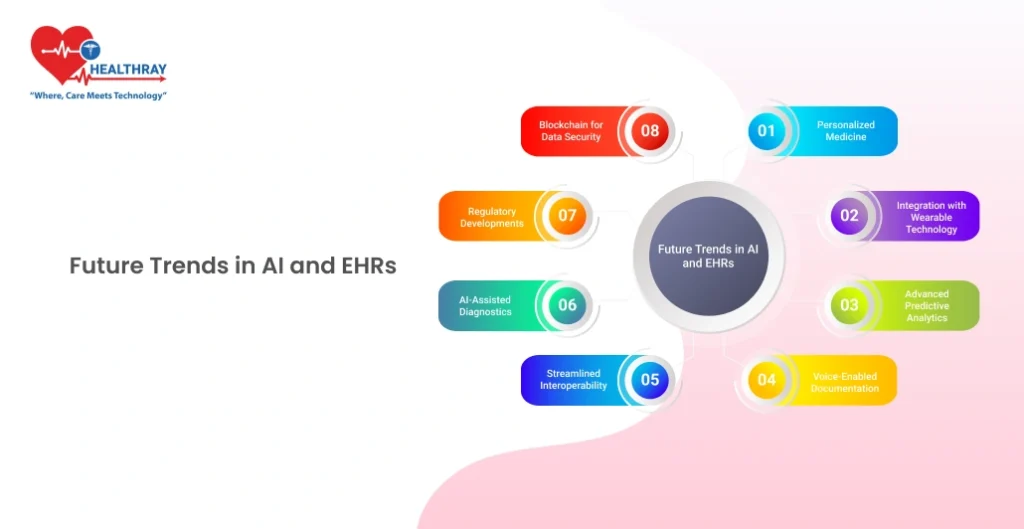
The integration of Artificial Intelligence (AI) with Electronic Health Records (EHRs) is just the beginning. The healthcare industry is poised for even more groundbreaking changes as technology continues to evolve. Here are the key trends shaping the future of AI and EHR systems:
Personalized Medicine
AI-powered EHRs are paving the way for personalized care by analyzing patient data at an individual level. From genetic profiles to lifestyle factors, AI can recommend tailored treatment plans, improving outcomes and reducing trial-and-error in care.
Integration with Wearable Technology
Wearables like fitness trackers and smartwatches are generating valuable health data. Future EHR systems will seamlessly integrate with these devices, providing real-time insights into a patient’s health. This can lead to early detection of conditions and better chronic disease management.
Advanced Predictive Analytics
AI will continue to enhance predictive analytics in EHRs. For instance, algorithms may soon be able to predict hospital readmissions, identify at-risk patients for certain diseases, or even recommend preventive measures based on community health trends.
Voice-Enabled Documentation
AI-driven voice recognition is making clinical documentation more efficient. Physicians can dictate notes directly into EHRs, saving time and reducing administrative burdens. Advanced natural language processing (NLP) will make these systems more accurate and intuitive.
Streamlined Interoperability
The future promises smoother data sharing between disparate systems. AI is helping to standardize data formats and bridge gaps between providers, ensuring that patient records are complete and accessible regardless of location.
AI-Assisted Diagnostics
AI-enhanced EHRs will integrate diagnostic tools that analyze medical images, lab results, and patient histories. These systems can flag abnormalities and suggest potential diagnoses, assisting healthcare professionals in making faster, more accurate decisions.
Regulatory Developments
Governments and regulatory bodies are actively working on policies to ensure safe and ethical AI use in healthcare. Future EHRs will need to comply with these regulations, shaping the way AI is developed and implemented.
Blockchain for Data Security
Blockchain technology may play a role in the future of EHRs by providing a secure and transparent way to store and share healthcare data. This could address longstanding concerns around data breaches and unauthorized access.
Conclusion
The evolution of Electronic Health Records, powered by Artificial Intelligence, is transforming healthcare. From improving data accuracy to enabling personalized medicine, AI is pushing the boundaries of what EHRs can do. Different types of EHR systems cater to various needs, whether it’s the robust control of server-based systems or the flexibility of cloud-based solutions.
However, implementing AI-enhanced Hospital Management Systems comes with challenges, including data security, interoperability, and cost concerns. By addressing these obstacles with practical strategies, healthcare providers and administrators can unlock the full potential of these technologies.
The future holds even greater promise. Innovations like wearables integration, advanced predictive analytics, and voice-enabled documentation are just the beginning. To remain competitive and provide top-notch patient care, embracing these changes isn’t optional—it’s essential.