Healthcare has always been at the forefront of technological innovation, and Electronic Health Records (EHR) are no exception. These systems have revolutionized how patient data is stored, accessed, and managed. But even with their widespread adoption, traditional EHR systems often face challenges like time-consuming data entry, incomplete records, and limited analytical capabilities. This is where artificial intelligence (AI) steps in, promising to redefine how EHRs work.
AI in EHR systems isn’t just about making processes faster; it’s about making them smarter. From automating routine tasks to offering predictive insights, AI enhances efficiency and reduces physician burnout. More importantly, it empowers healthcare providers with tools to deliver personalized and proactive care.
This article explores the many ways AI is shaping the next generation of EHR Softwares. We’ll dive into the benefits it brings, the challenges to overcome, and what the future holds. If you’ve ever wondered how AI is transforming healthcare from the inside out, this is the place to start.
Benefits of AI Integration in EHR
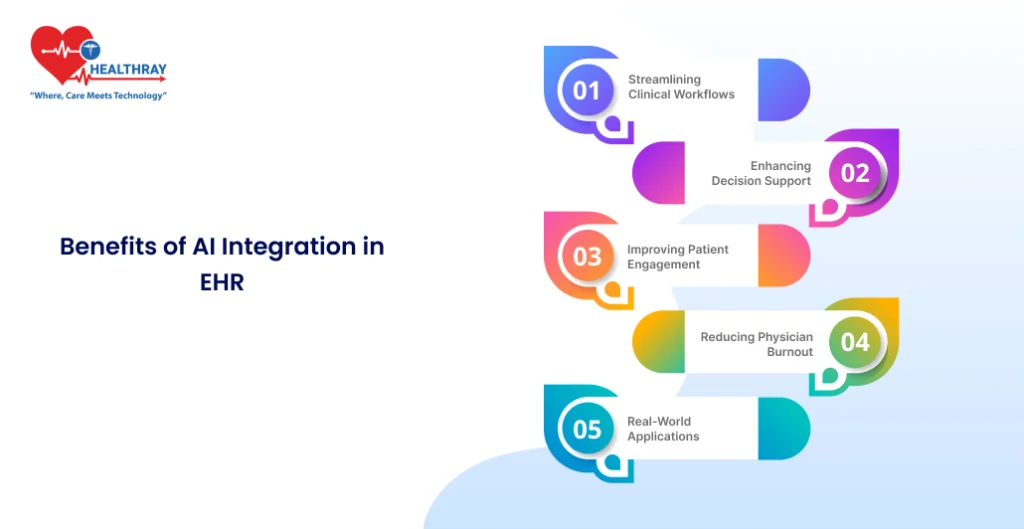
AI is transforming Electronic Health Records (EHR) into more than just digital filing systems. It’s making them smarter, faster, and more helpful for healthcare professionals. Let’s break down the key benefits.
Streamlining Clinical Workflows
AI takes the grunt work out of routine tasks. For example, it can automate patient appointment scheduling, billing processes, and even data entry. This reduces administrative workload, freeing up healthcare providers to focus on what truly matters—patient care.
Enhancing Decision Support
AI-powered EHR systems assist clinicians by analyzing patient data and alerting them to potential red flags. Whether it’s a dangerous drug interaction or a sudden change in a patient’s vital signs, these systems act as a second pair of eyes, ensuring nothing critical is overlooked.
Improving Patient Engagement
AI-driven tools help patients stay engaged in their healthcare journey. Chatbots can answer common questions, send medication reminders, or schedule follow-ups. Personalized patient portals also use AI to deliver relevant health insights and recommendations.
Reducing Physician Burnout
One of the biggest challenges for healthcare providers is the time spent on documentation. AI tools help by automating note-taking, simplifying coding processes, and reducing repetitive tasks. This not only saves time but also reduces stress for medical staff.
Real-World Applications
Several healthcare organizations are already reaping the benefits of AI-enhanced EHR systems. They report reduced errors, faster patient processing times, and improved satisfaction for both staff and patients.
AI-Driven Clinical Documentation
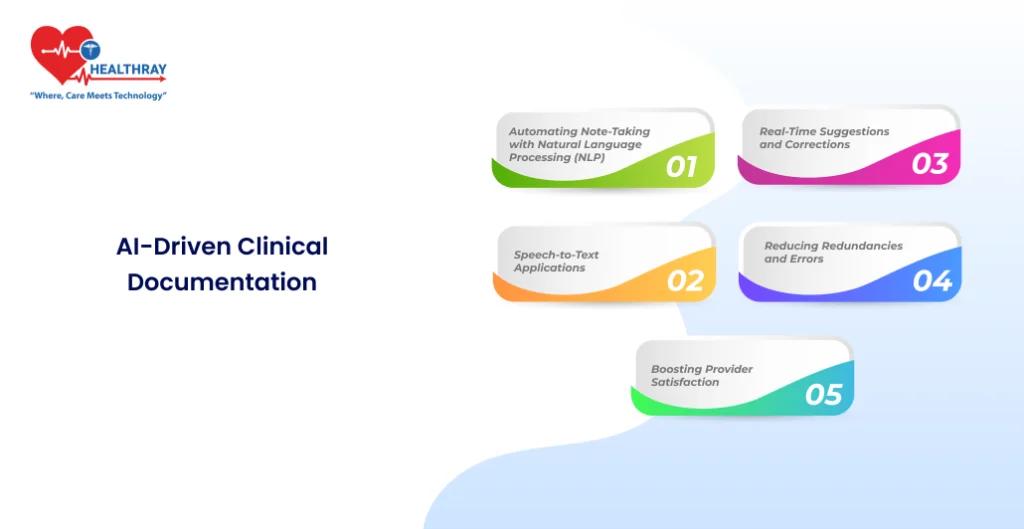
Documentation is one of the most time-intensive tasks in healthcare. It consumes hours that physicians could otherwise spend with patients. AI is transforming clinical documentation, making it faster and more accurate while reducing the administrative burden on healthcare professionals. Here’s how it’s changing the game.
Automating Note-Taking with Natural Language Processing (NLP)
AI-powered Natural Language Processing (NLP) tools are revolutionizing how medical notes are captured. These systems listen to doctor-patient conversations and convert them into structured notes. They identify key medical terms, diagnoses, and treatments, ensuring nothing important is missed.
Speech-to-Text Applications
With voice recognition technology, physicians can dictate notes directly into the EHR system. This eliminates the need for manual typing, saving time and reducing the risk of errors. AI ensures that the transcriptions are not only accurate but also contextually relevant.
Real-Time Suggestions and Corrections
Advanced AI tools go beyond capturing data—they analyze it. For instance, while a physician documents a case, AI can suggest appropriate diagnostic codes or flag inconsistencies. This helps maintain accuracy and ensures compliance with medical standards.
Reducing Redundancies and Errors
AI-driven documentation systems can identify and remove duplicate entries, reducing clutter in patient records. They also flag potential errors, such as conflicting medication details or missing information, ensuring high-quality records.
Boosting Provider Satisfaction
By automating tedious tasks, AI allows physicians to focus on patient interactions rather than paperwork. This shift not only improves job satisfaction but also enhances the overall quality of care delivered.
Predictive Analytics and Personalized Care
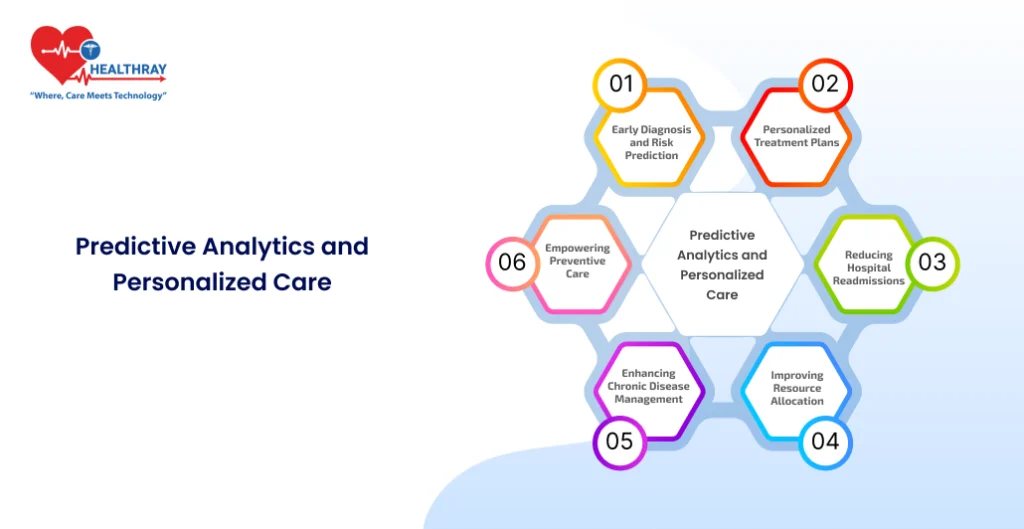
AI’s ability to analyze vast amounts of patient data is unlocking new possibilities for predictive healthcare and personalized treatment plans. Predictive analytics is not just about identifying trends; it’s about anticipating outcomes and taking action before issues arise. Here’s how it’s reshaping care delivery:
Early Diagnosis and Risk Prediction
AI algorithms analyze patient histories, lab results, and even genetic data to predict potential health risks. For example, AI can identify early signs of chronic conditions like diabetes or cardiovascular disease, often before symptoms become noticeable. This proactive approach allows for earlier interventions, improving patient outcomes.
Personalized Treatment Plans
AI ensures treatments are tailored to individual patients. By considering factors like genetics, lifestyle, and medical history, AI helps clinicians develop customized care plans. This level of precision not only enhances treatment effectiveness but also minimizes side effects.
Reducing Hospital Readmissions
AI-powered predictive tools can identify patients at risk of readmission after discharge. Based on patterns in patient data, clinicians can implement preventative measures, such as follow-up visits or medication adjustments, reducing the likelihood of complications.
Improving Resource Allocation
Hospitals can use AI-driven analytics to predict patient admissions and optimize staffing, bed availability, and resource allocation. This ensures the right resources are available at the right time, enhancing operational efficiency.
Enhancing Chronic Disease Management
AI tools monitor patients with chronic conditions in real-time, analyzing wearable device data and EHRs to provide insights. This continuous feedback helps healthcare providers adjust treatments dynamically, improving long-term outcomes.
Empowering Preventive Care
By identifying population health trends, AI supports preventive care initiatives. For example, public health agencies can use predictive models to prepare for flu seasons or outbreaks, ensuring better community health management.
Implementation Challenges and Solutions
The integration of AI into Electronic Health Records (EHR) comes with immense potential but also some significant hurdles. Successfully overcoming these challenges is essential for healthcare organizations aiming to harness the power of AI. Here’s a look at the main obstacles and practical solutions.
Data Privacy and Security Concerns
- Challenge: AI systems rely on vast amounts of patient data, raising concerns about compliance with privacy regulations like HIPAA. Unauthorized access or data breaches could compromise sensitive health information.
- Solution: Employ advanced encryption techniques, multi-factor authentication, and regular audits. AI tools should be designed with privacy by default, ensuring data is anonymized whenever possible.
Integration with Legacy Systems
- Challenge: Many healthcare facilities operate on outdated EHR platforms that aren’t optimized for AI integration. Adding AI tools to such systems can cause compatibility issues.
- Solution: Use middleware solutions to bridge the gap between old and new systems. Gradual migration to AI-friendly platforms can also help, ensuring minimal disruption to daily operations.
High Implementation Costs
- Challenge: Developing and deploying AI-driven EHR systems requires significant investment in technology, infrastructure, and training.
- Solution: Start small with pilot projects to demonstrate ROI before scaling up. Cloud-based AI services can also reduce upfront costs by offering pay-as-you-go models.
Resistance to Change
- Challenge: Healthcare professionals may resist AI adoption due to fears of job displacement or the complexity of learning new systems.
- Solution: Focus on training programs that highlight the collaborative role of AI, emphasizing that it supports rather than replaces clinical decision-making. Encourage feedback from staff to refine implementations.
Bias and Inaccuracy in AI Models
- Challenge: AI systems can unintentionally perpetuate biases if trained on incomplete or unrepresentative datasets, leading to inaccuracies in predictions or treatments.
- Solution: Use diverse and comprehensive datasets during AI model training. Regular audits and updates ensure models remain accurate and fair.
Regulatory and Ethical Concerns
- Challenge: AI in healthcare must comply with stringent regulations, and ethical dilemmas may arise regarding decisions made by AI systems.
- Solution: Collaborate with regulatory bodies during development to ensure compliance. Establish clear guidelines for how AI fits into clinical decision-making.
Ensuring User-Friendly Interfaces
- Challenge: Complex AI tools can overwhelm users, especially those unfamiliar with advanced technology.
- Solution: Prioritize intuitive, user-friendly designs that require minimal training. Interfaces should align with existing workflows to ensure seamless adoption.
Future Trends in AI and EHR
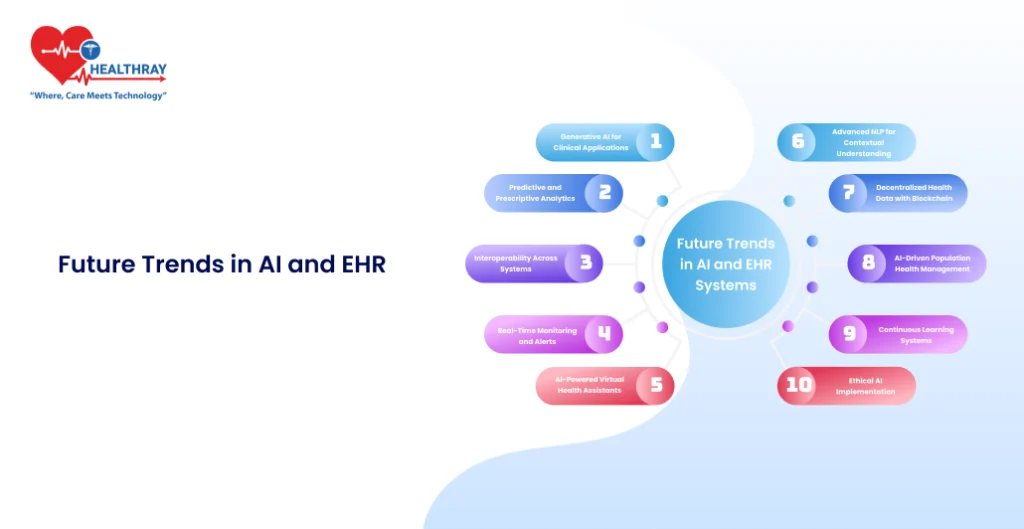
The future of Electronic Health Records (EHR) is being reshaped by the rapid advancements in artificial intelligence (AI). These innovations are poised to make EHR systems more intelligent, adaptive, and valuable for healthcare professionals and patients alike. Here are some trends to watch:
Generative AI for Clinical Applications
AI is evolving beyond basic automation to creative problem-solving. Generative AI models are expected to assist with drafting patient summaries, generating treatment recommendations, and creating individualized care plans. This will allow healthcare providers to spend more time on patient interaction and less on administrative tasks.
Predictive and Prescriptive Analytics
Predictive analytics has already started transforming healthcare, but prescriptive analytics is the next frontier. These systems won’t just forecast potential health issues; they’ll recommend actionable steps for prevention or treatment, tailored to individual patients.
Interoperability Across Systems
Future EHR systems will likely focus on interoperability, enabling seamless data exchange between different healthcare providers, devices, and platforms. AI will play a critical role in normalizing and integrating data from diverse sources, providing a comprehensive view of patient health.
Real-Time Monitoring and Alerts
Wearable devices and IoT sensors will work hand-in-hand with AI-driven EHR systems to offer real-time health monitoring. These tools will provide instant alerts for abnormal readings, allowing providers to intervene before conditions worsen.
AI-Powered Virtual Health Assistants
AI-based assistants are expected to play a bigger role in guiding patients and providers. For patients, these tools will offer medication reminders, symptom checkers, and health tips. For providers, virtual assistants will streamline tasks like scheduling, documentation, and diagnostics.
Advanced NLP for Contextual Understanding
Natural Language Processing (NLP) will become even more sophisticated, enabling AI to understand context in medical notes and conversations. This will enhance documentation accuracy and improve the way information is organized and retrieved.
Decentralized Health Data with Blockchain
AI and blockchain technologies will likely converge to create secure, decentralized health records. Patients may gain more control over their health data, deciding who can access their records and how.
AI-Driven Population Health Management
AI tools will enable healthcare systems to analyze population health trends more effectively. They will identify at-risk groups, predict outbreaks, and allocate resources efficiently, improving community-level healthcare.
Continuous Learning Systems
Future AI-powered EHRs will include continuous learning capabilities, improving their performance over time by learning from real-world data. This will enhance diagnostic accuracy and make predictions more reliable.
Ethical AI Implementation
As AI becomes more embedded in EHR systems, ethical considerations will gain prominence. Transparent AI algorithms and bias-free training data will become standard requirements, ensuring fairness and equity in healthcare delivery.
Conclusion
The integration of AI into Electronic Health Records (EHR) is more than a technological upgrade; it’s a paradigm shift in how healthcare is delivered. AI has the potential to transform EHR systems into tools that not only record patient data but actively contribute to better outcomes. From automating routine documentation to predicting health risks and personalizing treatment plans, the possibilities are vast.
However, this transformation isn’t without challenges. Issues like data privacy, system compatibility, and ethical considerations need thoughtful solutions. By addressing these, healthcare organizations can unlock the full potential of AI-driven Hospital Management Systems.
As the future unfolds, AI will likely become an inseparable part of EHRs, creating a healthcare ecosystem that is smarter, faster, and more focused on the patient. For providers, administrators, developers, and entrepreneurs, the time to explore and adopt AI-powered EHR solutions is now.