Electronic Health Records (EHR) have transformed the way patient data is managed in healthcare. However, challenges like manual data entry, limited interoperability, and administrative overload still persist. This is where Artificial Intelligence (AI) steps in. AI is reshaping EHR software by automating repetitive tasks, enhancing data accuracy, and supporting clinical decisions.
In this post, we’ll explore how AI is changing the game for EHR Softwares. You’ll learn about the key benefits, real-world applications, and what the future holds for healthcare powered by AI.
The Benefits of AI in EHR Software
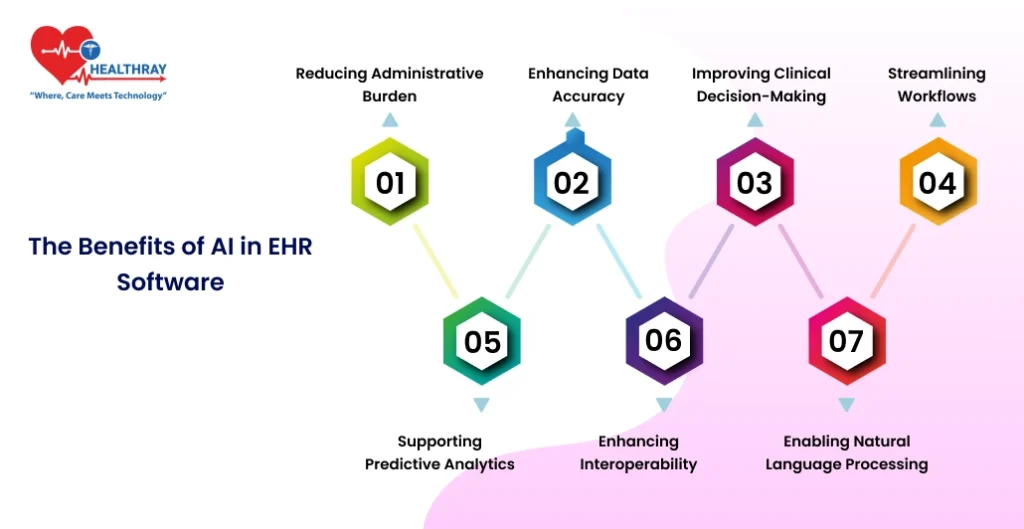
AI is revolutionizing Electronic Health Records (EHR) in several ways that directly address long-standing challenges in healthcare. Here’s how AI is making EHR systems smarter and more effective:
Reducing Administrative Burden
One of the biggest pain points for healthcare professionals is the time-consuming task of data entry. AI-powered tools can automate repetitive tasks like coding diagnoses, updating patient records, and managing billing information. This frees up more time for healthcare providers to focus on patient care.
Enhancing Data Accuracy
Manual entry often leads to errors that can impact patient outcomes. AI minimizes these risks by identifying inconsistencies in real time. It ensures that patient data remains accurate, complete, and up to date.
Improving Clinical Decision-Making
AI analyzes vast amounts of patient data to offer actionable insights. It helps clinicians identify patterns, predict potential complications, and recommend personalized treatment plans. This makes care more precise and patient-centric.
Streamlining Workflows
AI-driven EHR systems prioritize tasks based on urgency and relevance, helping healthcare teams stay organized. They also integrate seamlessly with other tools and systems, making the workflow smoother across departments.
Supporting Predictive Analytics
AI uses predictive models to anticipate health trends, detect disease outbreaks, and even predict individual patient outcomes. This allows healthcare providers to intervene earlier and reduce the likelihood of complications.
Enhancing Interoperability
Data silos are a persistent problem in healthcare. AI helps bridge gaps by integrating data from multiple sources, ensuring that healthcare professionals have a holistic view of the patient’s medical history.
Enabling Natural Language Processing (NLP)
Doctors often leave unstructured notes in EHRs, which can be hard to analyze. NLP tools powered by AI interpret this unstructured data, converting it into valuable insights that improve care and decision-making.
Challenges and Considerations in Implementing AI in EHR Systems
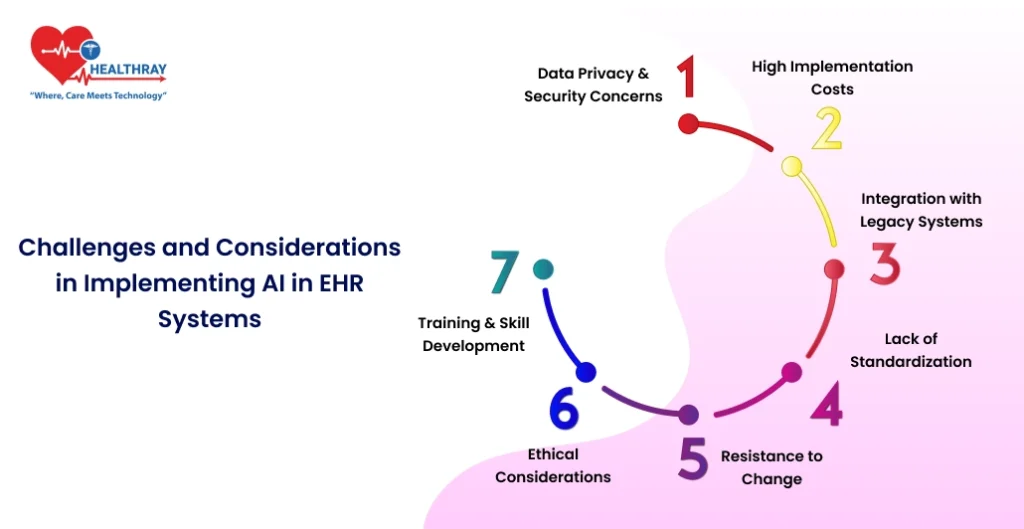
While AI holds immense promise for transforming Electronic Health Record systems, integrating it into healthcare comes with its own set of challenges. Here’s what healthcare administrators and decision-makers need to consider:
Data Privacy and Security Concerns
AI relies on massive amounts of patient data, making security a top priority. Unauthorized access or breaches can lead to severe consequences, including regulatory penalties and loss of trust. Implementing robust encryption and compliance measures, such as HIPAA, is essential.
High Implementation Costs
Deploying AI in EHR systems involves significant upfront investments in hardware, software, and training. For smaller healthcare providers, these costs can be a barrier. However, long-term savings from efficiency and accuracy may offset initial expenses.
Integration with Legacy Systems
Most healthcare facilities operate on legacy systems that may not easily integrate with AI-driven solutions. This can lead to compatibility issues and delays in implementation. Careful planning and phased rollouts can help mitigate this challenge.
Lack of Standardization
The absence of universal standards for EHR systems complicates the adoption of AI. Variations in data formats, workflows, and software requirements can hinder interoperability and slow down AI’s full potential in healthcare.
Resistance to Change
Adopting AI often requires a cultural shift within organizations. Clinicians and staff may hesitate to embrace new technologies due to fear of job displacement or skepticism about AI’s reliability. Clear communication and training programs can ease this transition.
Ethical Considerations
AI algorithms make decisions based on data, which can inadvertently introduce bias. Ensuring fairness and transparency in AI-driven systems is critical to avoid disparities in patient care. Continuous monitoring and audits are necessary to maintain ethical standards.
Training and Skill Development
Healthcare professionals need proper training to use AI tools effectively. Lack of familiarity can lead to underutilization of these systems. Organizations must invest in ongoing education and support for their teams.
Real-World Examples of AI in EHR Systems
AI integration into EHR systems is no longer a futuristic concept—it’s happening now. Here are some compelling examples that showcase how healthcare organizations are leveraging AI to improve patient care and operational efficiency:
Automating Patient Record Updates
Some hospitals use AI tools to automatically update patient charts by analyzing data from wearable devices and monitoring systems. This ensures that doctors have access to real-time information, helping them make informed decisions faster.
Enhanced Diagnostic Support
AI-powered EHR systems assist in diagnosing conditions by cross-referencing patient data with medical literature and global databases. For instance, Mayo Clinic has explored AI solutions that help identify rare diseases based on symptoms logged in EHRs.
Predicting Patient Readmissions
AI algorithms analyze EHR data to predict which patients are at higher risk of readmission after discharge. This allows care teams to intervene early with targeted follow-up plans, reducing hospital readmission rates.
Streamlined Prescription Management
AI is being used to flag potential drug interactions or dosage errors. This feature is particularly useful in high-volume hospitals where clinicians manage complex cases and multiple medications.
Natural Language Processing for Physician Notes
Healthcare providers like Stanford Health Care use AI-driven Natural Language Processing (NLP) to interpret unstructured data such as physician notes. This helps convert free text into actionable insights for better clinical decision-making.
Predictive Analytics for Population Health
AI-integrated EHRs are helping public health organizations predict and respond to disease outbreaks. By analyzing anonymized EHR data, AI tools can identify trends and enable preventive measures.
Virtual Assistants for Administrative Tasks
Virtual assistants powered by AI are reducing administrative workloads by scheduling appointments, retrieving patient records, and sending reminders. These tools free up human resources to focus on patient engagement.
The Future of AI in EHR Systems
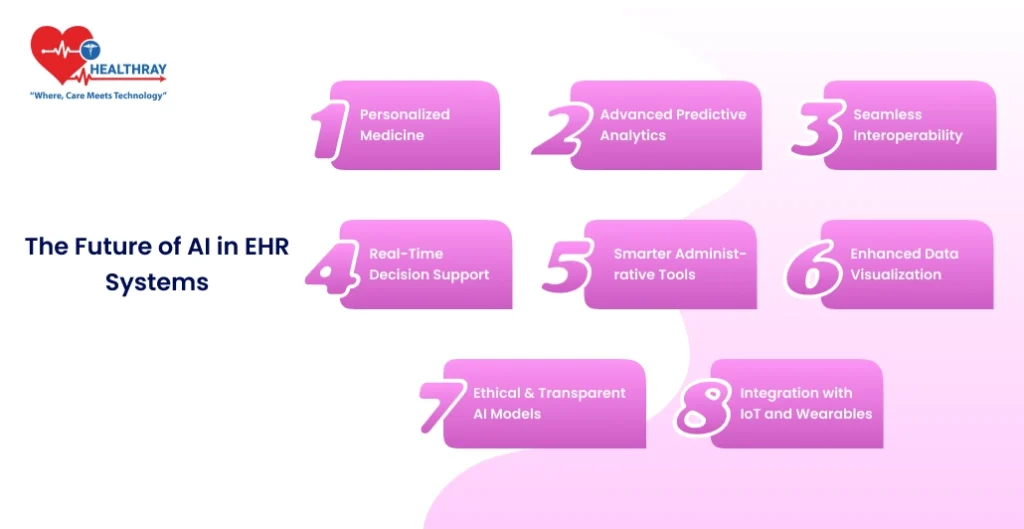
AI’s role in Electronic Health Records (EHR) is set to expand significantly, paving the way for a smarter and more connected healthcare ecosystem. Here’s a glimpse of what the future holds:
Personalized Medicine
AI will enable truly personalized healthcare by analyzing individual patient data to recommend treatments tailored to their genetic makeup, medical history, and lifestyle. This shift will make treatments more effective and outcomes more predictable.
Advanced Predictive Analytics
The next generation of AI tools will not only predict diseases but also recommend preventive strategies. For example, analyzing population health trends could help providers allocate resources more effectively and reduce the burden on healthcare systems.
Seamless Interoperability
Future EHR systems, powered by AI, will break down data silos by enabling seamless data sharing across healthcare facilities. This will give clinicians a comprehensive view of a patient’s health history, improving care coordination.
Real-Time Decision Support
AI will provide real-time insights during patient consultations. Tools like voice-enabled assistants could analyze conversations, update EHRs, and offer clinical suggestions instantly, improving the efficiency of every patient interaction.
Smarter Administrative Tools
As AI matures, administrative tasks like insurance claims processing and compliance monitoring will become fully automated. This will not only reduce costs but also improve turnaround times for critical operations.
Enhanced Data Visualization
AI will make patient data easier to interpret by providing visual insights like risk scores, trend graphs, and predictive models. This will empower clinicians to understand complex data at a glance.
Ethical and Transparent AI Models
The future will see the rise of explainable AI in EHR systems. This will allow healthcare providers to understand how AI makes decisions, fostering greater trust and adoption among professionals.
Integration with IoT and Wearables
AI-powered EHR systems will integrate more seamlessly with Internet of Things (IoT) devices and wearable tech. Real-time health monitoring and automatic data logging will enhance chronic disease management and preventive care.
Conclusion
AI is no longer a futuristic concept in healthcare—it’s transforming Hospital Management Systems today and promises even greater advancements tomorrow. By reducing administrative burdens, enhancing clinical decision-making, and improving patient outcomes, AI has become an essential tool for modern healthcare. While challenges like cost and data security remain, the benefits far outweigh the obstacles. For healthcare administrators, software developers, and decision-makers, adopting AI in EHR systems is not just an option; it’s a necessity for staying ahead in an evolving industry.